It feels like a holy war is brewing in data management. At the heart of these rumblings is something that may seem sacrilege to many data architects: the days of the traditional data warehouse are numbered. For good reason. As data volumes continue to grow exponentially, the industry is united in recognizing we need a faster, more agile way to leverage data to unearth insights and drive actions. But that’s about all the industry agrees on.
Battle lines are being drawn across ideologies and technologies. Each claim to be the silver bullet to solve the challenges data leaders and the businesses they serve face today. The hard truth? None are a panacea. All have their merits and drawbacks, and have drawn their fair share of supporters. In the leadup to an episode of The Data Chief LIVE, I polled data leaders to get their take - and as you can see, opinions were evenly divided.
The reader’s digest on the evolving world of data management
If you’re having trouble following all these different technologies, you’re not alone.
Here’s a quick primer:
The data warehouse:
The data warehouse has been a tried and tested concept going back to Bill Inmon’s first book in 1992, Building the Data Warehouse, and later, Ralph Kimball’s book The Data Warehouse Toolkit. While valuable for its time, a centralized data warehouse in an on-premises world could take months to build. Highly curated data can obscure more valuable granular insights. Costs could be high.
The data lake:
The term data lake was coined in 2010, with the promise of speeding access to granular data and lowering costs. Unfortunately, these became known as data swamps, too slow to be usable.
The data lakehouse:
The data lakehouse combines the best of both a data warehouse and a data lake, offering converged workloads for data science and analytics use cases. Databricks leverages this term in its marketing collateral, while Snowflake prefers the term The Data Cloud.
Data fabric:
Data fabric started as a particular concept in 2014 from NetApp. Since then, it has evolved conceptually and become a paradigm advocated by Gartner. The data fabric places a strong emphasis on metadata and AI to discover related data across cloud and on-premises data sets.
Data mesh:
Data mesh is both an architectural approach and organizational concept pioneered by ThoughtWorks and defined in Zhamak Dehghani’s new book Data Mesh: Delivering Data-Driven Value at Scale. In the data mesh, the end goal is a data product. Data is organized and controlled by domains, and should not be moved. For an excellent article on the data fabric and data mesh, check out Tony Baer’s article here, or check out this lively debate at Datanova.
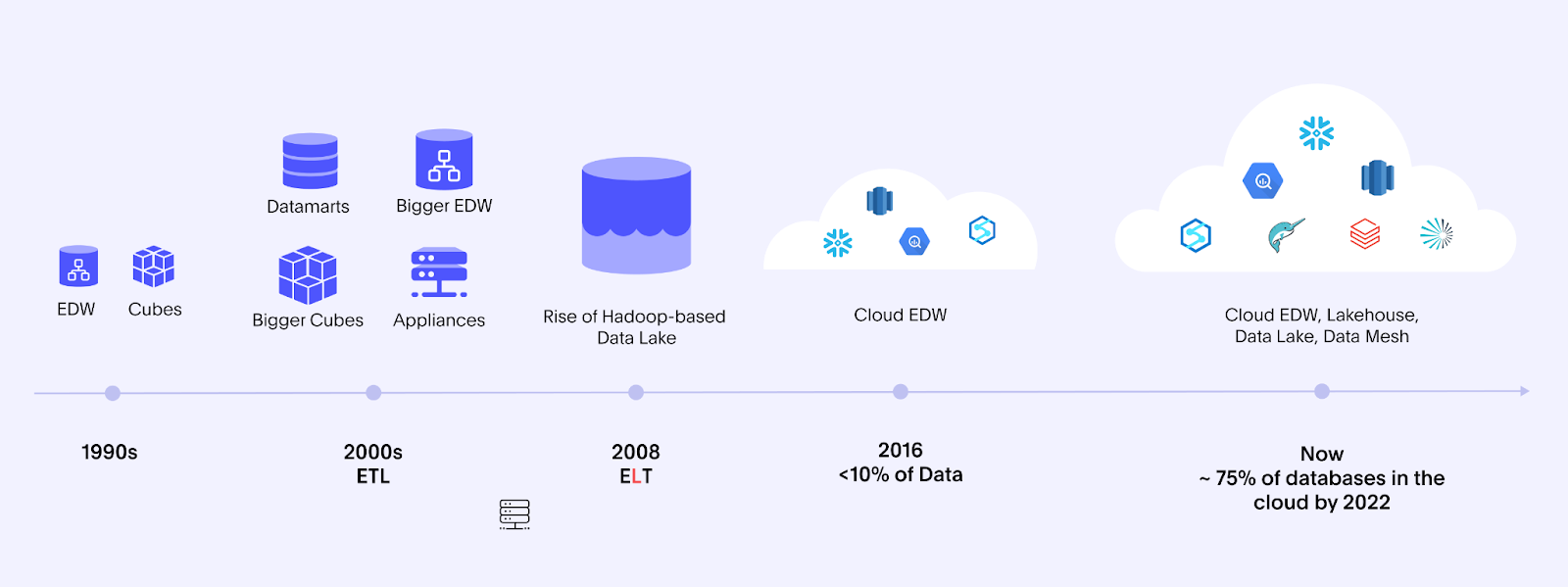
Haven’t we heard about the death of the data warehouse before?
This conversation may feel a bit like deja vu. It’s not the first time the industry has predicted the demise of the data warehouse as we know it. A decade ago in 2012, experts at the Strata-Hadoop World claimed that the data lake would kill the data warehouse. That death never materialized.
This time around, however, these predictions are grounded in broader trends that will finally mark the end of the traditional data warehouse. Newer concepts paired with technical innovations of cloud computing and converged workloads will finally dethrone the data warehouse of the past and make way for a more data-driven world.
This march of progress is not without it’s obstacles. Legacy processes and skills will be a critical barrier. This plays out when, for example, practitioners build cubes and aggregate tables in their cloud database, despite the benefits offered by other design approaches. Ensuring consistent master data will continue to be a requirement, regardless of the approach taken. Navigating these new concepts is the foundation your future digital success rests upon, and the time to embrace them is now.
To learn how, and see what other top trends are reshaping the world of data, check out our 7 data and analytics trends and predictions for 2024.