On a recent trip to New York City, I found myself with only 30 minutes to travel 2 miles between meetings. In the rain. In the past, this would have meant getting soaked while I walked (or ran) to a major intersection and tried to hail a cab. Now, I keep dry in the lobby for 3 minutes while I wait for an Uber.
Traditionally, we’ve relied on highly-trained specialists to get things done. Need a ride? Call a company to dispatch you one. Need to travel? Call your travel agent. Looking for a specific book? Talk to your librarian.
Specialization can be wonderful, but when it’s your only option it can also be limiting. Try hailing a cab in the rain if you don’t know what I mean.
AI Has Already Infiltrated Consumer Tech
In our personal lives, we’re already consuming more data than ever before. And we’re doing it, not just because there’s more data available, but because machine learning and AI have already become commonplace in consumer tech, making this data accessible. Experts still exist, but they’re increasingly specialized.
I won’t define machine learning and artificial intelligence here; there are many very good primers available if you’re interested in more information.
Instead of working with experts for everything, we’re increasingly following an “80/20 rule.” 20% of what we need still comes from experts – think of this as high-value, high-impact work. Scheduling complicated vacations, or finding that rare book written in 1810. Meanwhile, for 80% of what we need, experts have been replaced by technology that relies on AI and machine learning.
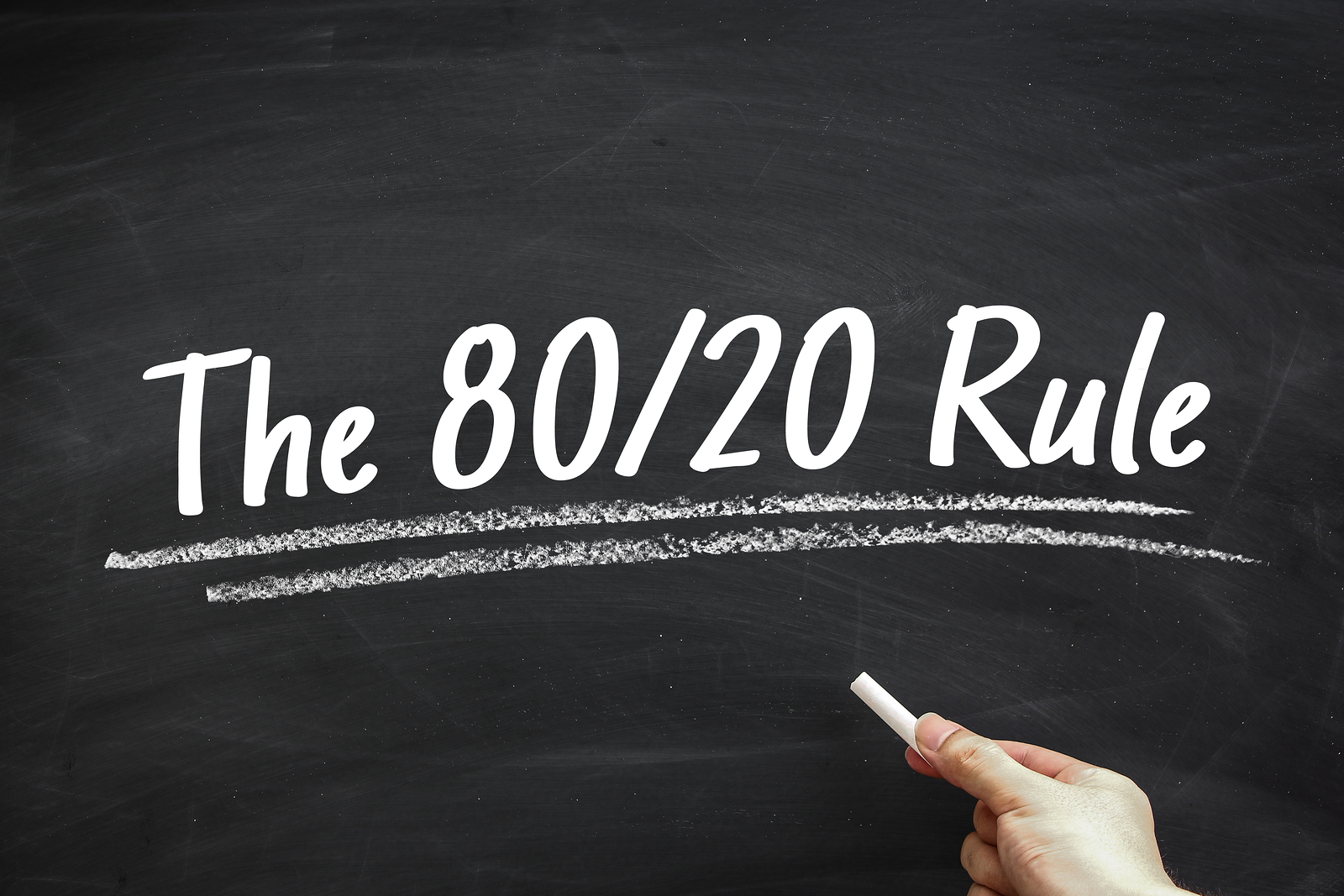
Instead of heading to the library, we pull up Google, whose machine learning algorithms not only provide relevant search results, but suggest answers before you type the question. Uber uses machine learning to dynamically manage supply, demand, and pricing based on many real-time factors, including what it knows about you, the rider. Spotify or Apple Music have replaced music critics, using machine learning and artificial intelligence to make recommendations based on our past behavior and people like us. And the list goes on, from using Netflix to watch a movie or asking Alexa what the weather will be like tomorrow, the consumerization of machine learning increasingly serves as our interface to information.
Enterprise Tech Takes its Cue
Of course, none of this has happened in our business lives when it comes to enterprise analytics software. At work, we still rely on experts to get most things done.
Access to information is a prime example. Data analysts don’t just focus on the 20% of the high-value, high-impact work; they are the only people doing the other 80% of analytics work that is routine and low-value, but also necessary. There’s a reason BI teams are often called report factories.
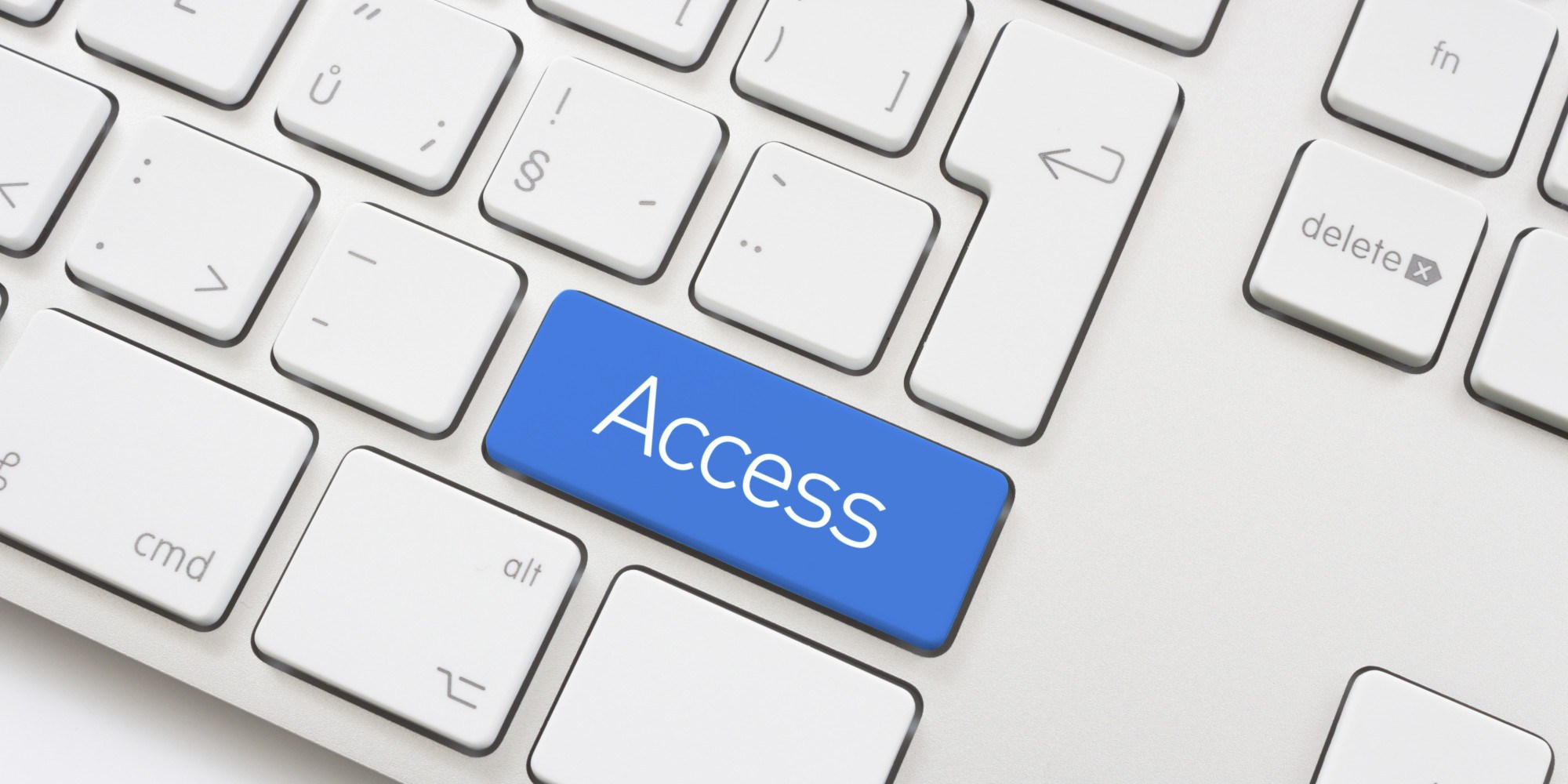
All of this is about to change, fortunately. We’re starting to see the same technologies that have revolutionized the consumer space make their way into business.
Companies like Bed Bath and Beyond, Chevron and Amway have already made analytics accessible by turning to a hallmark of consumer technology – search. The reason is simple—everybody already uses search every day at consumer sites like Google, Facebook, LinkedIn, and Amazon.
Things are starting to get even more exciting, though, with the introduction of next evolution technologies like machine learning and AI. The same specialization that has frustrated our attempts to introduce technology into our professional lives turns into a benefit here.
Because I’m interested in the same information and data at work, machine learning and AI will be able to learn about my usage, interests, typical queries and more. Understanding how I interact with data will allow AI to get smarter, to the point where it will know to answer questions I hadn’t even posed yet, in the same way Spotify knows to build a playlist I’ll love before I add even a single song.
That’s the promise of AI in the enterprise. And it’s now being realized.
The past decade of rapid technological advances have made it a golden time to be a consumer. Some of that magic is finally rubbing off on our business lives.
I’m looking forward to the decade ahead.
Originally published on inside BIGDATA.