The way we interact with AI is changing fast.
Forget chatbots that need a million prompts to produce anything halfway useful. With agentic AI, you get autonomous agents that handle the grunt work before you even think to ask.
Just look at NVIDIA. They launched Agent Morpheus, an AI framework that uses real-time data processing to automatically detect threats and keep security airtight. It’s the kind of setup that bridges the gap between thought and action, letting teams zero in on what actually moves the needle.
For teams like NVIDIA’s, agentic AI isn’t just another tool—it’s the invisible force that keeps everything moving without a hitch. Ready to see how it can work for you? Let’s dive in.
Table of contents:
- What is agentic AI?
- How can agentic AI reshape the way you work?
- What are the most impactful agentic AI examples & use cases today?
- Sales teams: Accelerating lead conversion
- Security teams: Proactive threat detection
- Development teams: Faster code delivery
- Product teams: Smarter product roadmapping
- Marketers: Real-time campaign optimization
- Support teams: Better experience for your customers
- Business users: Instant, actionable Insights
- Operation teams: Agile business operations
Agentic AI is a type of artificial intelligence that can think, learn, and act on its own without requiring constant human input. These autonomous AI systems demonstrate core capabilities like reasoning, memory, and autonomous decision-making to solve complex, multi-step problems. Much of this intelligence is powered by the multimodal capabilities of generative AI and advanced foundation models.
Unlike typical chatbots that require specific prompts, these agents learn from data, process real-time information, and execute tasks with varying degrees of human oversight.
Think of it as the leap from passive assistance to proactive execution.
Increased productivity: Think about all those repetitive tasks you do daily—like sorting emails, pulling reports, and tracking metrics. They’re necessary, but a total time drain. Agentic AI quietly takes these tasks off your plate, allowing you to focus on the work that actually drives results.
Personalized workflows: Imagine a system that understands your workflow inside-out. Agentic AI acts like your personal assistant, helping you with all your routine tasks, like suggesting the best time to send a campaign or prioritizing urgent tasks. It’s all about working smarter, not harder.
Proactive decision-making: You can’t always predict the future, but with smart AI agents, you get better at anticipating it. By analyzing real-time patterns, it lets you forecast demand, prep for peak periods, and catch shifts in customer behavior before they impact your bottom line.
Better collaboration across teams: Most teams struggle to stay on the same page. Insights live in one tool, tasks in another, and priorities shift constantly. Agentic AI connects the dots by bringing shared insights to the surface, assigning follow-ups, and helping teams move as one.
Smarter suggestions: The more you use AI agents, the smarter they get. It learns your habits, adapts to your workflows, and continuously fine-tunes its support, delivering smarter insights and spot-on suggestions with every interaction.
From handling everyday tasks to driving greater agility across cloud solutions, Agentic AI use cases are reshaping the way work gets done across every industry.
Your sales team’s time is too valuable to waste on cold leads. The faster they zero in on the right prospects, the quicker your pipeline moves.
Modern intelligence tools make that possible by giving reps the insights they need, right when they need them:
Qualify leads automatically: Highlights the prospects most likely to convert, so your team can focus on deals with real potential.
Serves personalized content: Provides tailored follow-up responses, so reps know exactly what to say and when to say it.
Flags at-risk deals: Spots deals that are at risk of losing momentum and suggests targeted actions to keep them moving down the pipeline.
As your business grows, the need for actionable insights only intensifies. With ThoughtSpot's Agentic Analytics Platform, your team doesn’t have to wait for analysts. They can jump straight into the data, ask follow-up questions, and get real-time answers, keeping every lead and opportunity in sight.
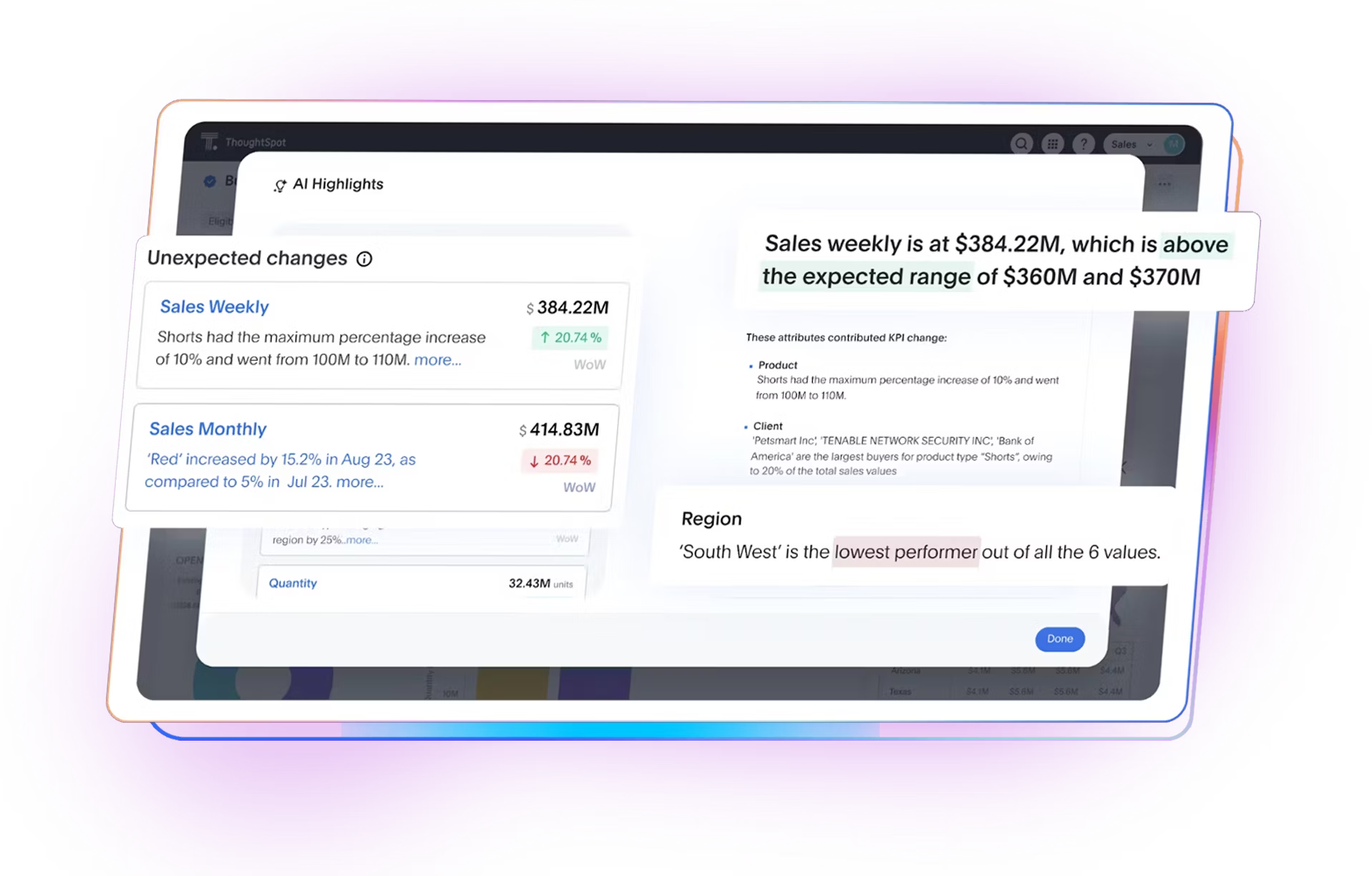
A McKinsey report found that it takes organizations an average of 73 days to contain a security breach—a glaring sign that most security systems are too slow to keep up.
Static defenses aren't built for today’s threat landscape. You need an intelligent layer that adapts, learns, and acts in real time. That’s where smart AI agents step in:
24/7 network monitoring: Constantly scans for anomalies, triggering automated responses to contain threats before they escalate.
Simulated attacks: Run proactive drills to identify vulnerabilities before attackers do.
Behavioral analysis: Score user activity in real time, helping your team focus on genuine threats instead of low-priority alerts.
This kings of intelligence doesn’t just strengthen your defenses, it reengineers your entire security system to become faster and more resilient.
Shipping code shouldn’t be so stressful. But let’s be real—manual reviews and endless bug fixes can easily drain your team’s momentum.
No wonder Gartner predicts that by 2028, nearly 90% of enterprise software engineers will rely on AI code assistants. And with agentic AI, you get a vigilant co-pilot that can handle the repetitive stuff that eats up your day:
Codebase scanning: Detects inefficiencies before they escalate into bigger problems.
CI/CD Monitoring: Tracks build processes in real time, catching errors before they derail schedules.
Bug prioritization: Flags critical issues first, so your team stays focused on high-impact fixes.
With most of the heavy lifting off their plate, your developers finally get to do the work: build cool things, move fast, and stay focused on the work that matters.
Want to turn your app into a smart app? With ThoughtSpot Embedded, you can infuse advanced AI analytics into your internal or customer-facing applications, turning every interaction into a source of actionable insights:
When every stakeholder wants their feature prioritized, roadmapping becomes a tug-of-war. It’s tough to know what truly deserves attention, and even harder to back those decisions with data.
However, with the right agentic AI solution, your product team can finally cut through the noise to:
User behavior analysis: Gather qualitative data from user research, feedback, and surveys, giving you a holistic understanding of customer pain points. This gap analysis helps you create smoother customer experiences.
Churn risk detection: Spot features linked to churn, helping you pivot resources to what actually matters.
Competitive tracking: Monitors shifts in competitor strategies, allowing you to refine your roadmap and stay competitive.
Goodbye, roadmap chaos. With the right AI analytics tools, you can stay on top of customer expectations and the competitive landscape to make smarter, data-backed decisions on what to build next.
Marketing teams are juggling more than ever—shifting user behavior, tight deadlines, fragmented channels, and a mountain of analytics tools. Personalizing interactions and optimizing campaigns in real time often feels out of reach.
Here’s how AI-powered tools bring clarity to the chaos and drive real outcomes:
Adapt messaging in real-time: Adjusts campaigns based on real-time user interactions, showcasing relevant content to the right user at the right time.
Refine audience segments: Targets high-value audiences, from repeat purchasers to high-engagement users, maximizing ROI.
Predict user actions: Anticipates what content users will engage with next and adjusts content to drive better conversions.
While AI handles the data crunching and tactical pivots, your team can focus on what truly moves the needle—creative strategies that truly resonate with your audience.
It’s no secret that support teams are stretched thin. Every new ticket, follow-up, or repeated issue only adds to their backlog. And at the end of the day, the slow response times only leave your customers more frustrated.
AI-powered support agents help you break that cycle by shifting your team from reactive to proactive:
Prioritize tickets by urgency: Triage and route tickets based on urgency, sentiment, and product behavior, allowing you to focus on the most critical issues.
Catch problems early on: Detects system anomalies and opens tickets automatically, sometimes before users even notice an issue.
Keep the help center relevant: Updates documentation based on trending questions, empowering users to find answers faster.
Armed with this clarity, your team can focus on solving complex issues and delivering a support experience your customers expect.
We know that finding those ‘aha’ moments in your data is challenging, especially when you’re working with static dashboards and outdated reports. And when you want to dig deeper, you're often stuck waiting weeks for your data team to get back to you.
Imagine having a data analyst who never clocks out. That’s what agentic AI use case in analytics feels like.
With AI tools like ThoughtSpot’s Spotter, you don’t have to rely on clunky dashboards anymore. These smart, adaptive systems automatically surface the insights, visuals, and summaries you need. Basically, you have the freedom to explore your data from any angle, anytime.
Even better, it understands your goals, analyzes data on the fly, spots trends, and recommends what to do next. What used to take weeks now takes seconds.
Every manual process, inefficiency, and delayed decision carries a price. The ability to quickly dig into the data and see exactly what’s eating into your bottom line. What your ops teams really need is agility, not more spreadsheets.
Luckily, with the right agentic AI solution, you can do just that—and more:
Forecast demand: Predicts supply needs and triggers inventory reorders automatically, so you’re never caught off guard.
Optimize shipping routes: Adjusts delivery routes in real time based on traffic, delays, or costs, keeping your shipments on schedule.
Monitor suppliers: Tracks supplier performance and flags risks, preventing production slowdowns before they happen.
This proactive shift turns your operations into a predictive engine, saving you time, money, and headaches.
💡 Unlock your AI edge: When you really get what each type of AI does best, you’re not just using a tool—you’re putting it to the right task. Ready to see how? Here’s your complete guide.
From static dashboards to agentic analytics
To drive measurable results with Agentic AI—or any AI initiative, for that matter—you can't settle for just another chatbot or basic chart generator. You need analytics that can handle the complexity, security, and scale of your enterprise data to deliver actionable insights right where it matters.
Meet ThoughtSpot’s AI Analyst, Spotter. It delivers decision-ready insights and enterprise-grade experiences, empowering you to tackle the most complex questions across massive, real-world datasets.
And that’s just the beginning. Spotter is just one part of our Agentic Analytics Platform. With features like Analyst Studio and automated insights, you can turn every interaction into an opportunity to grow your business.
The future of BI is here, and it’s powered by agents. Are you ready to join us? Request a demo today!