A few years ago, I received a call from the Tesla service team advising me not to come for my car service—a savings of $600 and my time. Imagine my surprise. In over two decades of car ownership, no auto service had ever called me and asked me not to show up.
The Tesla representative explained that the data from my car prescribed an action, or in this case, an inaction. By using data to save me a trip to the dealership, Tesla was able to create a positive user experience and gain a loyal customer—a strategy that generates more lifetime value than the $600 I would have paid for unnecessary maintenance.
The picture this story paints is even more relevant today. As data and AI become more infused in our day-to-day lives, we’re normalizing the efficiencies it brings and adapting to new ways of living, working, and conducting business. It’s no longer enough to be a data-driven digital business. To truly get ahead, you need to be an AI-driven business. That’s why at ThoughtSpot, we say AI is the new BI.
The promise of AI agents is paving the way toward that autonomous, AI-driven future. In the proceeding article, we’ll examine what it means to be an autonomous business and define what an AI agent in analytics should be like—discussing core capabilities and exploring the many benefits.
Table of contents:
At this point, we all know that the analysis of data and information followed by action results in better value chains. Better, in this case, is defined as efficiency, productivity, and customer experience. Companies that leverage business data and information to make better decisions have a competitive advantage over those that do not.
Over the last three decades, the data and analytics industry has made strides in enabling and expanding data-driven decision-making. These incremental improvements largely coincide with broader technology innovations. Consider how computing (1960s), internet (1990s), cloud computing (2000s), mobile computing (2010+), and AI (2020+) have enabled every industry to rapidly transform.
In the graphic below, you can see how these technology shifts overlay with the innovation in data and analytics. Throughout each technology cycle, we’ve gained additional types of analytics—descriptive, diagnostic, and eventually predictive. This chart represents the digital business era, a time where individuals are using data to make decisions.
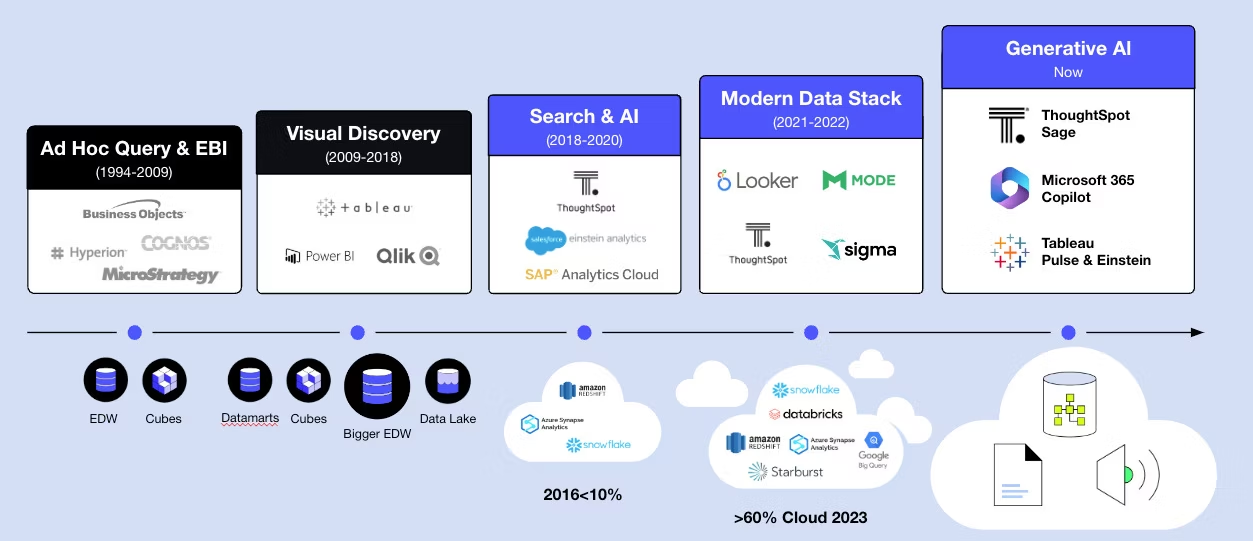
Generative AI is poised to fundamentally reshape the way we interact with data. So what can we expect from the post-digital-business era? Gartner characterizes the next macro phase of technological change as the autonomous business operating in a programmable economy.
What is autonomous business?
Autonomy is the next step in the AI evolution. First, AI gave us predictive analytics using machine learning. Then, we saw the rise of assistive—think chatbots and copilots. And now, we’re looking towards autonomous.
An autonomous business is one that has evolved from human-led and technology-assisted decisions to AI-initiated, human-supervised actions. Think about your day-to-day tasks. How might you be able to automate efficiency, productivity, and customer experiences across your business and operations?
Artificial intelligence (AI) agents are software programs created to autonomously perform a specific task based on a goal, with input or context. For example, an AI agent, also referred to as an AI assistant or agentic AI system, might be able to determine the best questions to ask for an objective to be met, route between two potential paths, decide which tools to call, or assess whether a generated answer is sufficient.
With GenAI-powered natural language understanding, there is an AI assistant being built for every role within a business. You’ve likely already encountered AI agents in some form of customer service, incorporating natural language processing to understand and respond to customer inquiries or answer questions. AI agents can also be used to automate recurring tasks that require little to no human intervention.
While the long-term potential uses are endless, I believe the AI assistants will initially help us remove human-powered mundane and tedious tasks from our workflows, enabling us to focus on human intelligence (HI). AI and HI will collaborate to make businesses a lot more productive. This applies to all areas of the business including product, marketing and sales, customer support—and of course, analytics.
Analytics must evolve to support the speed and automation required by modern businesses—transforming from predictive and prescriptive to true autonomy with varying degrees of human intervention and oversight. That means that AI agents will be able to evaluate data and context, ask questions, and make decisions within the parameters of human control.
While this may sound far out or even a bit unnerving for some, I find it helpful to think about this in more simplistic terms. Think back to my Tesla example, and consider an AI agent calling me to tell me I could skip my routine service appointment rather than a human customer service representative. The AI agent may be able to answer some of my questions, but I would be able to interact with a customer service representative in the event I requested one.
Within this context, you can easily see how the autonomous business is within reach for those digital businesses who have already invested in their data infrastructure and set their business up for data-driven decision-making. However, the benefits of automation and AI agents for analytics far exceed the realm provided by data-driven decision-making alone.
Process large volumes of data quickly
Imagine you’re operating a retail company with customer data scattered across various platforms—say website interactions, in-store purchases, social media engagement, and customer service records. With legacy BI tools, gathering and analyzing such massive data volumes could take weeks, even months. That’s because legacy BI tools often require manual data processing along with a “file a ticket and build a dashboard in weeks/days” type of methodology, creating potential bottlenecks at each step. Imagine running your business with dashboard-ticket drudgery, while competing against those empowered with instantaneous, AI-powered answers to questions as they arise.
AI agents, on the other hand, instantly pull data from any source, process it, and generate actionable insights within minutes. These smart, adaptive systems are designed to scan billions of rows of data and detect inconsistencies, ensuring that clean and high-quality data is available for further analysis. With a solid, reliable data foundation, your team can focus on what matters most—finding actionable insights and driving business growth.
Focus on outcomes with speed of insight-to-action
We know that finding ‘aha’ moments in your data is challenging, especially when you’re working with static dashboards and outdated reports. These traditional tools often provide a snapshot of data at a specific point in time, restricting your ability to fetch real-time insights. And let’s say you do find a point of interest—perhaps a sudden spike in customer engagement or a dip in sales. Finding the cause often requires another request to your data team. The result? Missed opportunities, slower responses to market changes, and an inability to make data-driven decisions in real-time.
AI agents are a game-changer. Instead of building dashboards from the ground up, you can use AI agents to surface relevant insights, visualizations, and text summaries. These intelligent systems understand your business goals and KPIs, analyze your data on the fly, uncover trends and spot anomalies, and even take the recommended actions—a feat that could have taken weeks to complete using a legacy dashboard.
Improve data accessibility for everyone
Picture this: Your sales team is working hard to track deals as they move through various stages of the pipeline. But they’re relying on a mix of spreadsheets, CRM tools, and dashboards—each filled with data points, charts, and figures that are difficult to piece together. To uncover valuable insights, they must wade through a maze of tabs, reports, and scattered data, wasting precious time.
Now, imagine a simpler way. With the right AI agent for analytics, anyone can simply type a question in plain English and receive meaningful insights. That’s right—no more relying on overburdened data teams to build a new dashboard for every query. You can effortlessly query data in natural language and instantly receive the answers you need, without requiring any technical expertise. This seamless access to crucial insights empowers everyone in your organization to make quicker, smarter decisions.
Streamline and automate decision-making
Building on your LLM’s adept training and your company’s expansive data, AI agents can be trained to autonomously perform various decision-making tasks without requiring constant human oversight. Think back to the Tesla example: if you have the data and the LLM in place, you can train your agent to analyze the data based on a trigger—perhaps that’s within the scheduled maintenance window. You can train the agent on what to look for. In this case, analyzing specific data points to see if the car needs maintenance. And finally, you can train the agent on what actions to take in the event that the agent encounters data within each specific parameter, such as sending an automated email or having a customer service bot call the customer to cancel the appointment.
These systems are designed to adapt, learn, and improve continuously, becoming more efficient and effective over time. As they process more data, AI agents refine their understanding of patterns, trends, and business objectives, allowing them to make increasingly accurate predictions and decisions. This evolving autonomy not only streamlines decision-making but also frees your team from tedious report-building and repetitive tasks—enabling organizations to scale and innovate more rapidly.
Uncover anomalies and opportunities
Think about seasonal patterns, new product launches and product promotions, natural weather disasters, or let’s say, a pandemic—all of these examples can have an impact on human behavior, and therefore your data. Traditional statistical methods often fall short when identifying unusual patterns within erratic data. That’s because these methods rely on rule-based programming and predefined parameters, which are not designed to adapt to the constantly evolving nature of complex datasets. As a result, valuable insights are overlooked and businesses struggle to respond quickly to emerging market trends or unforeseen shifts.
In contrast, AI agents are flexible and dynamic, capable of digesting new information and continuously adjusting their models to detect subtle anomalies. For instance, an AI agent could quickly spot a sudden dip in customer engagement during a typically high-performing season, whereas traditional methods might miss this until it's too late. This adaptability allows businesses to swiftly pivot their strategies, respond to market changes, and make data-driven decisions—all in real time.
Improve data team productivity
Data teams have got a lot on their plate. From building and training predictive models to constantly monitoring KPI changes, they are swarmed with operational tasks that often fall outside of their core responsibilities. On top of that, they frequently face ad-hoc requests for reports, insights, and data visualizations from different departments, adding even more to their already heavy workload.
With AI agents, data teams can finally get the support they need to stay ahead. Agents can initiate and manage time-consuming tasks such as data logging, data processing, real-time monitoring, and trend analysis. With such enhancements, instead of spending all their time measuring others’ progress, data teams can focus on important initiatives and drive big business outcomes, such as boosting ROI from investments or reducing waste in the value chain, fostering a data-driven culture, and being a coach to AI in order to improve business operations.
We predict that 2025 will be the year of the AI Analyst—the AI agent built specifically for enterprise-scale analytics. However, for the data and analytics industry to fully capitalize on this opportunity and deliver reliable, accurate results, the AI agent must evolve to embody the core competencies of a human analyst.
Business user perspective
An AI analyst should function much like any skilled analyst at your organization, helping business users understand data, ask the right questions, and receive accurate answers. To assess if an AI agent can truly embody a business user's perspective, here are some questions to ask yourself:
Natural language: Does the agent communicate in natural language and provide the context business users are familiar with?
Goal driven: Does the AI agent help a business user start from a goal, help ask and answer the right questions, and then lead them to recommended actions?
Data literacy: Can the AI agent help a business user understand and explore data by providing suggestions? Does it break down complex elements within the data through easy-to-understand tables, columns, and charts?
Query literacy: Does it guide business users in framing the right questions for data analysis? Can the AI agent suggest meaningful questions or queries related to the topic being explored?
Breadth and depth of questions: Is the AI agent capable of answering a wide range of analytical questions? Can it handle broad queries (like ‘What happened?’) and more complex ones (e.g., ‘Why did this happen?’)? Does it have the ability to generate formulas or insights for more advanced questions?
Accuracy and explainability of answers: What processes are in place to guarantee the accuracy of the AI agent’s responses? How does the agent clarify the methodology behind its calculations, and is the explanation clear and verifiable by a business user?
Multi-turn conversation: Can the analyst support multi-turn conversation and retain the context, while offering alternatives to improve accuracy?
Personalization: How well does the AI agent personalize recommendations and rankings for individual users, taking into account their role, department, and organizational affinity?
Data and Analytics team perspective
For the analyst and the data teams, the following key considerations are essential to ensure your AI agents meet enterprise-grade standards and deliver consistent, reliable results:
Trainability: How effectively can you train the AI agent to understand the business language and data taxonomy specific to your enterprise? Given that public language models are trained on general information, which technology stack is needed to bridge this gap?
Governance: Can data teams establish clear governance frameworks to ensure the AI agent adheres to compliance standards?
Safety: Given that the AI agents process natural language inputs, what steps should you put in place to address safety, ethics, and bias concerns? How can the responses be constrained to prevent hallucinations or the introduction of bias?
Time to value: As human analysts focus more on driving business outcomes, how can we make the AI agent training process more efficient? Which steps can reduce the need for direct involvement from human analysts?
Security: Which security measures can you implement to ensure your data, operations, and suggested actions are secure and compliant with your organization’s and industry’s standards?
Reliability: What mechanisms are in place to ensure the agent consistently delivers reliable results, even as it adapts to evolving data inputs? How can the system maintain and improve accuracy over time?
Technology perspective
When implementing AI agents, several technical factors come into play. Addressing these factors will ensure that your AI agents deliver accurate scalable results, while also being flexible enough to adapt to evolving business needs.
Multi-data system: Given that enterprise data is often fragmented across cloud data warehouses, databases, files, apps, and on-prem systems, how effectively can the AI agent integrate and analyze data from these various sources?
Multi-LLM (Foundation model choice): In what ways does the AI agent offer flexibility in selecting and certifying different Large Language Models (LLMs) for business needs? Does the agent allow you to maintain control over the LLM being used?
Agentic skills: Does the technology stack behind the AI agent support the rapid evolution of AI capabilities? How can you design the AI agent to improve results and learn new skills?
User experience
When it comes to adopting AI agents, an intuitive user experience is critical in ensuring that business users can easily interact with the technology and derive actionable insights. Here are key considerations for delivering a seamless and effective user experience:
Self-service: Is the UI intuitive enough for the business users to ask follow-up questions and drill down for more details?
Discover and explore insights: Does it encourage exploring existing analytical Insights?
Simplicity for advanced analytics: Is the user experience straightforward and designed to provide accurate, verifiable answers, while also integrating advanced data techniques? How well does it accommodate business users with different levels of data literacy?
Visualization capabilities: Can the AI analyst automatically generate and display the most appropriate chart based on the question being asked?
Satisfy curiosity your way (converse, click, or code): Does the AI analyst offer the flexibility of a BI platform? Can it allow users to ask follow-up questions or drill down into visualizations for granular insights?
Available in your workflows
Data and insights should be accessible where users already are—in their workflows, within applications, and on the go. To make sure your AI agents add value to your users without disrupting their workflows, consider these key points:
In-app: Is the AI agent available within your analytics app, either as an add-on or built-in feature?
Standalone: Can the AI agent be used on its own, without needing the full analytics app, for users who just want to ask data questions?
Embedded: Can the AI agent be integrated as a feature within other tools or applications?
Mobile: Is the AI agent easily accessible through a mobile app? Does it allow users to ask questions and receive answers on the go?
Insights to action
The true power of data analytics lies not just in uncovering insights, but also using those insights to make smart, timely decisions and take decisive action. To ensure that your AI agent is well-equipped to help you achieve this vision, consider the following:
Automate follow-up: Does the AI agent allow users to ask follow-up questions, retrieve answers, and set automated anomaly alerts whenever necessary?
Actionable: Can users instruct the AI analyst to take action once the analysis has been reviewed?
Whether we’re ready or not, AI agents are paving the way to autonomous businesses. How you prepare for and adapt to this evolution will depend on the readiness of your data, the people you have in place, and the viability of the technology you bring into your business. When it comes to data and analytics, don’t settle for just another chatbot or chart generator.
Bring your data to life with Spotter, ThoughtSpot’s AI agent for analytics—the intelligent AI Analyst that learns with you. Spotter is specifically designed to meet the complexity, security, and scale of enterprise data, with the usability and transparency that makes AI accessible to everyone.
We hope you’re as excited about the AI evolution as we are. Today, I’m inviting you to join us and try Spotter for yourself today.