Imagine a world where the mundane tasks associated with preparing, analyzing, and reporting data are handled by smart systems that learn and evolve. This isn’t science fiction—it’s the new reality of augmented-data management. In this new era, your data isn’t just processed—it’s actively managed by systems that seamlessly clean, integrate, and enhance it in real time.
Augmented data management systems proactively engage with your data, ensuring every piece is accurate, enriched, and ready for action. It’s like having a team of data wizards working tirelessly behind the scenes, transforming complexity into clarity and perfectly presenting your data according to your needs.
Table of contents:
Augmented Data Management (ADM) refers to the use of artificial intelligence (AI), machine learning (ML), and automation to streamline data handling. Unlike traditional data-management methods, which often depend on manual inputs and rigid protocols, ADM introduces a layer of smart, adaptive systems that continuously learn and evolve.
More than a mere upgrade from legacy software, ADM transforms how data is processed and analyzed for insights. By automating routine tasks and applying intelligent algorithms, ADM not only speeds up data processes but also verifies their accuracy and enhances their relevance. With ADM, you spend less time on repetitive tasks and more time focusing on strategic decisions that drive your business forward.
For example, imagine a retail company that uses ADM to manage its inventory. Typically, inventory management involves automated tracking and forecasting based on historical data. However, AI and ML can improve these processes by identifying real-time insights and more accurate predictions, transforming inventory management from basic automation to a sophisticated, data-driven approach.
With ADM, an AI-driven system can automatically track inventory, predict stock shortages or surpluses, and adjust orders accordingly. It learns from sales trends, seasonal variations, and even external factors like market changes and supply chain challenges to ensure inventory levels are always optimized. This AI-powered process saves time, reduces errors, and improves stock availability, leading to a more efficient and profitable operation.
Enhanced data quality and accuracy: ADM systems can automatically clean and enrich data. By deploying AI algorithms, these systems identify inconsistencies, missing values, and errors more efficiently than manual processes can. These enhancements ensure data is accurate and up-to-date.
Streamlined data integration: Manually combining data from various sources can be complicated, but ADM simplifies this task through automated data integration. AI-powered tools can intelligently map, merge, and harmonize data from disparate systems, making it easier to gain a unified view of your organization’s operations.
Improved data governance: With augmented management, data governance is proactive rather than reactive. Intelligent systems can monitor data usage, ensure regulatory compliance, and enforce data policies, reducing the risk of data breaches and legal liability.
Faster insights and analytics: By automating routine data tasks, ADM accelerates the time it takes to generate insights. Advanced augmented analytics tools like ThoughtSpot use ML to swiftly analyze large volumes of data, uncover trends, and deliver actionable insights that drive business decisions.
ThoughtSpot’s SpotIQ automatically sifts through data to reveal hidden patterns and correlations. Use SpotIQ to generate forecasts and explore various possibilities with what-if scenarios.
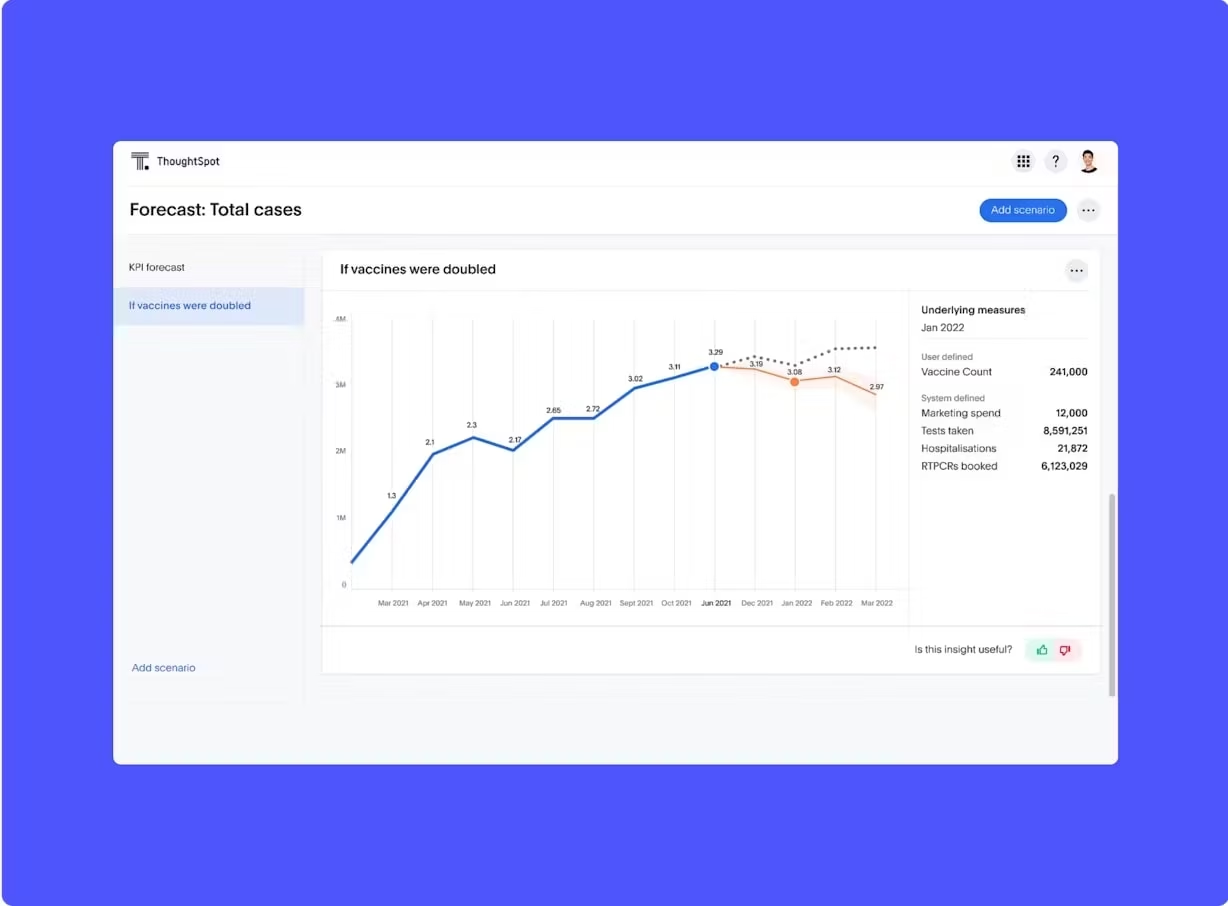
Adaptive learning and evolution: One of ADM’s most exciting aspects is its ability to learn and adapt. As the system processes more data, it improves its algorithms, making future data management tasks even more efficient. This continuous learning loop prevents data hallucinations and biases, ensuring your data management practices evolve with your business needs.
ADM involves several augmented intelligence tools and processes. These elements work together to streamline data handling and enhance the overall efficiency of data-driven decision-making. Let's break down the core components:
1. Automated data preparation:
One of ADM’s foundational aspects is the automation of data preparation tasks, which involves:
Data cleaning: Identifying and correcting errors or inconsistencies within the data such as duplicate entries, incorrect formats, or missing values. ML algorithms can detect patterns in data-quality issues and apply appropriate corrections.
Data transformation: Converting data into the desired format or structure, making it compatible with different systems or analysis requirements. This process includes data normalization, standardization, and aggregation.
Data enrichment: Augmenting data with additional information from external sources such as demographic data, geolocation, or third-party data sets to provide richer context for analysis.
2. AI-powered data integration:
Integrating data from various sources—whether they are cloud-based, on-premises, structured, or unstructured—is a complex task augmented data management simplifies through:
Intelligent mapping: Automatically identifying relationships and mappings between data sets—for example, matching customer records from different systems—to create a unified data view.
Data federation: Allowing access to data from different sources without the need to physically move or replicate it, thereby providing real-time access to up-to-date information.
Data virtualization: Creating a virtual layer that consolidates data from multiple sources, allowing users to query and analyze data without needing to know its physical location or format.
3. Real-time data monitoring and compliance:
Augmented data management tools provide continuous monitoring of data quality, integrity, and usage. Key components include:
Anomaly detection: Using AI to detect unusual patterns or outliers in data, which could indicate errors, fraud, or other issues. Real-time anomaly detection helps organizations respond swiftly to potential problems.
Compliance monitoring: Ensuring data management practices adhere to relevant regulations and standards such as GDPR or HIPAA. ADM maintains automated compliance checks and audit trails to reduce the risk of non-compliance.
Data lineage tracking: Providing a detailed history of data, including its origins, transformations, and usage. This transparency helps organizations understand how data flows through their systems and ensures accountability.
4. Advanced analytics and reporting:
With the foundational tasks streamlined, ADM focuses on delivering actionable insights through:
Predictive analytics: Leveraging ML models to forecast future trends and outcomes based on historical data. These analytics can include predicting customer behavior, sales trends, or risk factors.
Natural Language Processing (NLP): Enabling users to interact with data using natural language queries and making complex data analysis accessible to non-technical users. Tools like ThoughtSpot allow users to ask questions in plain English and receive visual answers, simplifying the reporting process.
Data visualization: Creating intuitive, interactive, and visual data representations makes it easier to identify patterns, trends, and insights. Examples include dashboards, charts, graphs, and more tailored to specific business needs.
5. Data security and privacy:
Ensuring data security and privacy is a critical ADM function. Components include:
Data encryption: Protecting sensitive data through encryption, both at rest and in transit, to prevent unauthorized access.
Access control: Implementing robust access control measures, including role-based access controls and user-authentication mechanisms, to ensure that only authorized individuals can access or modify data.
Data masking and anonymization: Hiding or altering sensitive information, making it difficult to identify individuals or personal details, thus protecting privacy.
1. Financial services
The financial industry is a prime beneficiary of ADM due to its reliance on vast amounts of data for decision-making, risk management, and regulatory compliance. ADM systems enhance fraud detection by using ML algorithms to identify unusual transaction patterns, thus protecting customers and financial institutions alike.
Additionally, these systems enable more accurate risk assessments by analyzing market trends and customer data, providing valuable insights for investments and loan approvals.
Loan Market Group faced challenges with inflexible reporting tools in their MyCRM system. After implementing ThoughtSpot Everywhere, the company enhanced reporting capabilities with a user-friendly, interactive interface, leading to a revamped experience for over 4,000 users. This shift improved user engagement and operational efficiency.
2. Healthcare
In the healthcare sector, efficient data management is crucial for improving patient care and outcomes. ADM facilitates the integration of electronic health records (EHRs) and other patient data, providing healthcare providers with a comprehensive view of patient histories. This comprehensive data access improves diagnosis and treatment planning.
ADM tools also support clinical decision-making by analyzing patient data to provide real-time insights and recommendations, such as identifying potential drug interactions or suggesting alternative treatments. Moreover, ADM aids public health monitoring by tracking disease outbreaks, vaccination rates, and other health indicators, thereby informing timely interventions and policy decisions.
Wellthy, a healthcare consulting company, faced issues with slow, manual reporting using legacy tools. By adopting ThoughtSpot, it replaced static reports with interactive Liveboards, boosting its analytics team’s output by 281% and reducing costs by $200K. This shift enhanced real-time data exploration and client interactions.
“There's so much work that goes into data from the backend—modeling, transforming, building out dashboards, producing insights, and so on. But, if data isn’t being used to make a decision, then you're missing the most important part, right? The last mile is key.”
3. Retail
The retail industry leverages ADM to enhance customer experience and optimize operations. By analyzing customer data such as purchase history and browsing behavior, ADM systems enable personalized marketing strategies and product recommendations, increasing customer engagement and boosting sales.
ADM also plays a crucial role in inventory management, predicting demand trends and optimizing stock levels to reduce costs and prevent overstock or stockouts. Additionally, it can analyze market trends, competitor pricing, and consumer behavior to refine product positioning and pricing strategies, helping retailers maximize profitability while staying competitive.
In the early days of the pandemic, Canadian Tire adopted ThoughtSpot to streamline inventory management, leading to a 20% rise in sales while 40% of their physical stores remained closed.
4. Hospitality
The hospitality industry benefits significantly from ADM solutions, which enhance operations and improve employee engagement. By leveraging analytics, hospitality businesses can streamline human capital management, optimize recruitment processes, and boost operational efficiency.
Harri, a leader in human capital management, adopted ThoughtSpot Everywhere and SpotIQ to enhance its platform–Harri iQ. This advanced analytics integration empowered managers with intuitive, actionable insights, improving recruitment and operational processes. The solution enabled Harri to adapt to labor market demands, secure new contracts, and enhance decision-making.
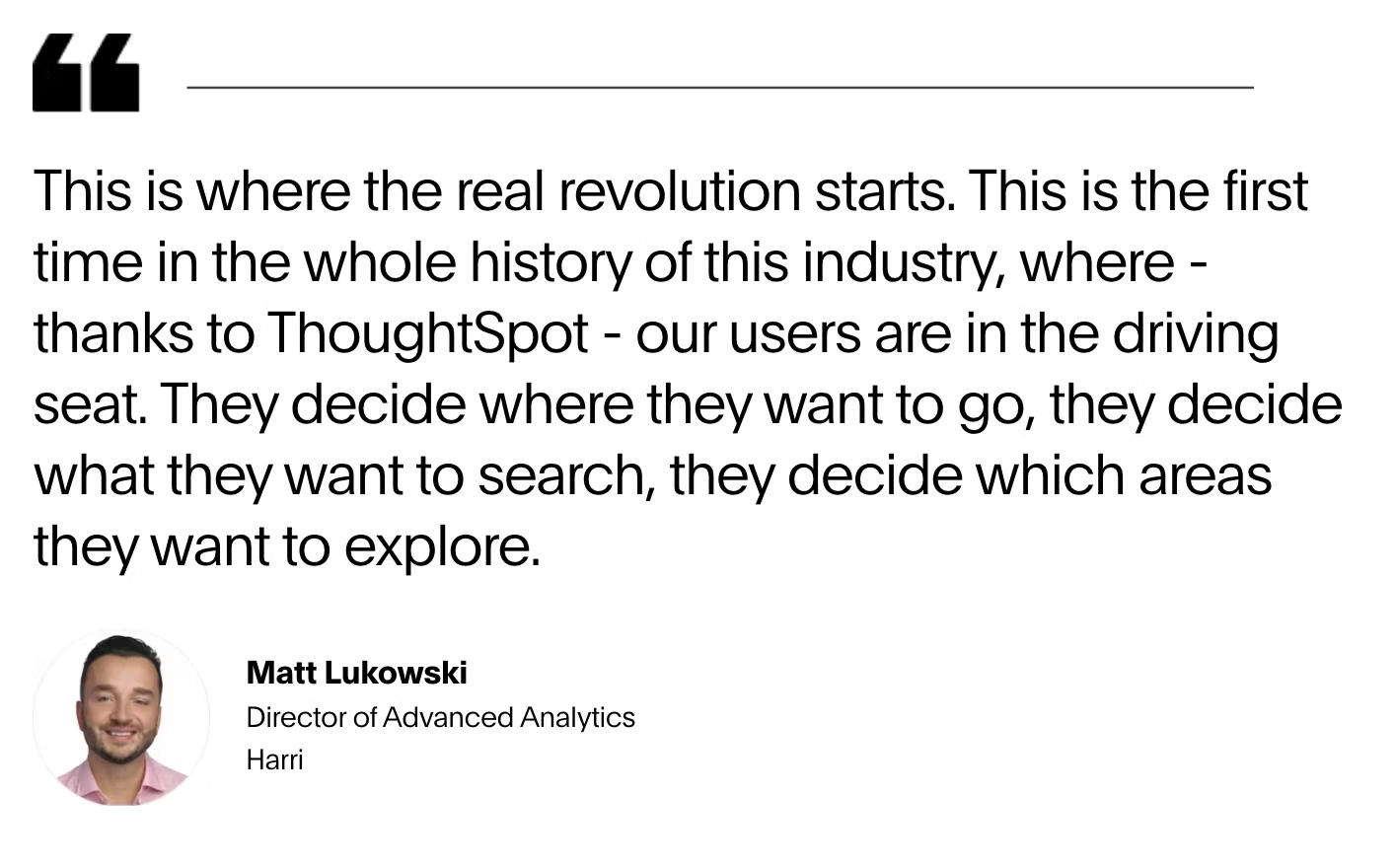
To leverage ADM to its fullest, it is essential to select the right set of tools. A solution that incorporates advanced AI and automation not only simplifies data management but also enhances the speed and accuracy of insights.
ThoughtSpot is setting new standards for how businesses leverage their data. By integrating AI and natural language search, ThoughtSpot enables users to explore and analyze data in exciting new ways.
Discover how ThoughtSpot can elevate your data management practices and provide deeper, more actionable insights with ease.
With ThoughtSpot’s AI-Powered Analytics, you can seamlessly interact with your data through natural language search.
Design interactive Liveboards and receive personalized insights with SpotIQ.
Features like Change Analysis and AI Highlights use advanced algorithms for real-time insights. Change Analysis helps users identify change drivers and perform root-cause analysis, while AI Highlights provides swift insights into key metric changes, helping you focus quickly on what matters most.
ThoughtSpot offers AI-assisted data modeling and exploration tools, along with Sage Coach, to streamline complex data tasks and enhance accuracy.
Stay informed with automated alerts on key KPIs through ThoughtSpot Monitor, ensuring you’re always up-to-date with important metrics.
Access your data and insights from anywhere with ThoughtSpot’s mobile app, keeping you connected and informed no matter where you are.
ThoughtSpot ensures data integrity and protection with centralized governance controls, verified Liveboards, and comprehensive security features.
Upgrade your data strategy with ThoughtSpot and unlock new possibilities for your business — take a product tour today.