As their data volumes grow, it becomes increasingly difficult for organizations to understand when and why their data changes. Ask any analyst to uncover the root cause behind KPI variability and it could take them weeks—or even months—to come back with an answer. That’s because most legacy business intelligence (BI) tools require data teams to spend an inordinate amount of time on remedial tasks instead of actual data analysis. From manually aggregating data from disparate sources to managing complex dashboard updates, this endless cycle makes real-time decision-making nearly impossible.
Luckily, Artificial Intelligence (AI) is powering a new generation of data-driven decision-making. By combining big data and AI, you can now uncover insights from massive datasets in a matter of minutes, making it more important than ever to understand how these two technologies can work in tandem.
Table of contents:
Big data refers to extremely large volumes of structured, unstructured, and semi-structured data that grow rapidly over time. These datasets are so massive that traditional processing methods often struggle to handle them efficiently. But AI-powered big data analytics tools can efficiently examine these complex datasets to uncover meaningful insights, patterns, trends, and correlations.
Challenges with big data
Big data is your company’s most valuable asset, but managing, analyzing, and interpreting it isn't easy. Here are the five most common big data challenges most businesses face:
Data volume: When you’re collecting terabytes or even exabytes of raw data from multiple sources, managing and storing such vast information becomes incredibly complex. Without a solid data warehouse architecture and the right tools for extraction, business users and analysts can’t easily access and retrieve the information they need.
Data variety: With data scattered across emails, documents, and spreadsheets, finding qualitative insights from quantitative data can be extremely challenging. This fragmentation hampers the ability to effectively leverage data for analysis and decision-making.
Data veracity: Big data is often hard to organize, making it difficult to maintain consistent data quality and accuracy. Without reliable data, you risk getting a distorted view of your business, which can lead to confusion and inaccurate insights.
Data security: With cyber threats on the rise, implementing robust security measures to safeguard sensitive information is crucial. However, the sheer volume of confidential data makes it difficult to manage access controls while maintaining compliance standards.
Data velocity: In practical terms, the nature of big data is continuously evolving and expanding. But to generate meaningful insights, it’s crucial to process this ever-increasing information flow in real-time, which in turn, requires a lot of time and manual plumbing.
AI is a set of technologies that allow computers to perform a wide variety of functions, including generating images, giving natural language responses, analyzing data, making recommendations, and more. This broad field includes machine learning (ML), natural language processing (NLP), robotics, and deep learning that help computers and systems reason, learn, and act intelligently.
From collecting vast amounts of data to identifying patterns and relationships, AI is reshaping every phase of data and analytics workflows. AI-powered big data analytics helps businesses solve big data problems and accelerate the speed at which insights are generated. Let’s look at how this works:
1. Handling large data volumes at scale
With legacy BI tools, organizations are so busy preparing and modeling big data, it can take a considerable amount of time to go from collected data to insight to action. In fact, reports show that 92% of data workers spend their time siphoned away by operational tasks outside of their roles, leaving very little time to actually analyze data.
Modern data analytics solutions like ThoughtSpot are designed from the ground up for enterprise-scale analysis. By leveraging big data and AI, ThoughtSpot can easily comb through billions of data rows and execute dozens of insights-detection algorithms — all within seconds. For example, you can connect ThoughtSpot to a cloud data warehouse like Snowflake or Databricks and gain instant insights into your cloud data.
Here’s how ThoughtSpot connects with Snowflake and empowers businesses to unlock the true value of their data using natural language search and AI-powered insights:
2. Easy follow-ups via natural language processing (NLP)
Generative AI has brought us to a place where the available technology can actually deliver on the promises that self-service BI has made for the last decade. Instead of basic dashboards or static reports, we have a more intuitive, conversational way of interacting with their data.
Natural language processing (NLP) is a powerful machine learning ability that makes it easier for anyone to ask questions in everyday language—no complicated code required. Simply type a question and get meaningful, actionable insights. This self-serve experience allows users—even those without technical expertise—to ask the next question and the next until they can fully understand the data behind a metric.
See in action how ThoughtSpot's AI-driven search interface empowers users to effortlessly query their data and gain real-time insights within seconds:
3. Enterprise-grade governance
Big data doesn’t guarantee results unless the data is accurate, relevant, and properly protected. Because these datasets are varied and complex, it can be harder to implement data governance policies and controls to protect them.
Today’s AI-powered solutions like ThoughtSpot provide complete transparency where you need it most. With granular controls built into every workflow and comprehensive audit logs, you have end-to-end visibility into who’s accessing what. What’s amazing is you can even see and understand how NLQ and AI-driven insights are translated into structured query language (SQL) with visuals showing how each answer was generated.
4. Automatically surfaces consumable and relevant insights
Every business leader believes big data is the key to understanding what’s happening in their organization. They even think analyzing such data can help them pinpoint the reasons behind any changes or anomalies. Unfortunately, this is easier said than done.
One of the most exciting benefits of combining big data and AI is its ability to help you dig in and understand the specifics of when, where, and why. These features make it easier to tell a powerful data story that drives business outcomes. For example, ThoughtSpot’s SpotIQ uses machine learning to uncover meaningful drivers behind KPI changes. It automatically explores to discover insights on your behalf, finding outliers you didn't even think to look for.
Companies are leveraging the power of big data and AI across industries to optimize processes and drive meaningful outcomes. New use cases are being developed every day, showcasing just how impactful these technologies can be. Here are a few examples:
1. Advertisement industry:
AI-powered big data analytics tools can help you understand how different campaigns resonate with your customers. By analyzing vast amounts of user engagement data such as click-through rates, view time, and interaction patterns, advertisers can quickly identify which campaigns aspects are performing well and which need adjustments. These insights will help you make informed decisions related to boosting customer experience through messaging, targeting, and budgets.
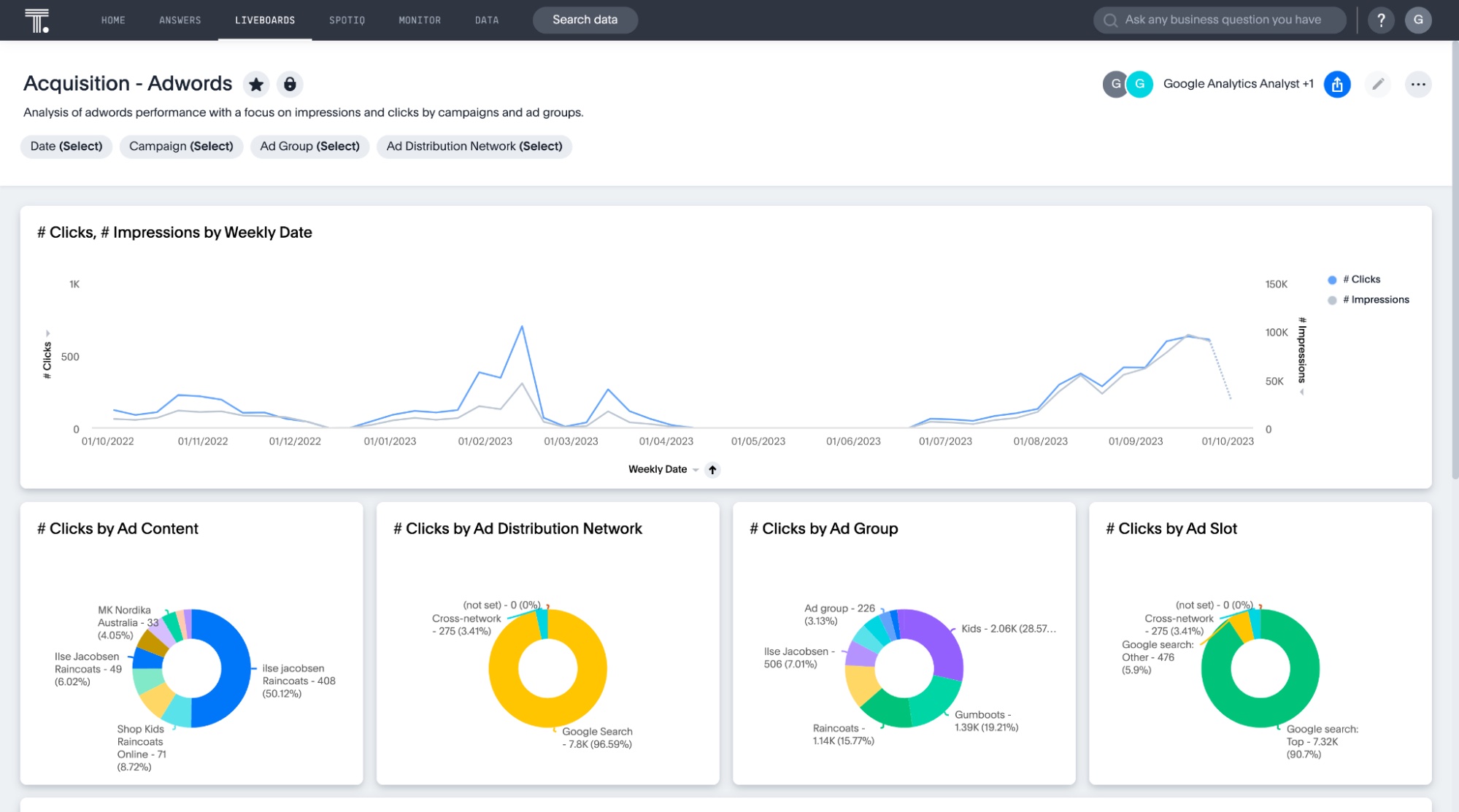
2. Retail industry:
In the fast-paced world of retail, big data and AI are helping businesses optimize their operations, particularly pricing and inventory management. Retailers can evaluate the impact of pricing changes on sales, understand demand fluctuations, and predict future buying patterns. By integrating AI-driven predictive analytics, companies can optimize stock levels to avoid overstocking or stockouts, enhancing efficiency and customer satisfaction while reducing costs.
See how Candian Tire was able to boost sales by 20%, even as 40% of their brick-and-mortar stores temporarily closed during the height of the COVID-19 pandemic.
3. Healthcare:
By analyzing vast data from electronic health records, diagnostic reports, and wearable devices, healthcare providers can analyze readmission rates and uncover key factors impacting patient health. These data-driven insights allow clinicians to create tailored treatment plans, resulting in more precise and effective care.
AI data analytics is designed to support, automate, and simplify each stage of your big data analysis journey. However, you can’t simply implement a solution and expect it to immediately solve all your problems. Issues such as hallucinations and biases in AI can undermine even the most well-intentioned efforts. Here are some expert tips to ensure your AI analytics investments reap maximum ROI:
Align your generative AI strategy with business goals: Create a well-defined outline of how your business intelligence investments will drive decisions and decide on a timeline for deployment. It’s also crucial to calculate ROI and understand the risks.
Always allow human oversight: While AI works as an autonomous system, human oversight remains crucial to maintain the accuracy of results. It’s important to have a human-in-the-loop element embedded in your BI and data analytics strategy.
Implement a measurement framework: Develop a robust system for tracking the return on investment (ROI) from your generative AI efforts. Ensure your framework captures both short-term wins and long-term gains.
Upskill your business teams: Launch a training program aimed at helping business users gain the skills to analyze data. These upskilling programs will boost adoption rates and allow teams to discover new use cases.
Invest in the right analytics tools: Evaluate different BI platforms based on their capabilities, ease of use, and compatibility with existing systems. The right tools should empower users to interact seamlessly with data, enabling them to derive insights quickly and efficiently.
💡Important note: Keep in mind that when it comes to leveraging big data with AI, there is no one-size-fits-all strategy. What works for one company might not be the right approach for your organization’s specific needs.
GenAI has invigorated the promise of true self-service analytics, which—when combined with enterprise-scale governance—can empower you to quickly understand and act on your data insights to make a tangible impact.
Recognized as a leader in Gartner MQ for analytics and BI, ThoughtSpot delivers real-time data experiences through features like Monitor, SpotIQ, and drill-anywhere visualizations. Our patented search technology closes the chasm between humans and machines, bringing insights directly to users while allowing them to endlessly explore their data until their curiosity is satisfied.
Take the first step towards unlocking the power of your big data with AI-Powered Analytics—request a ThoughtSpot demo.