Artificial intelligence (AI) is delivering impressive wins for businesses like yours—helping them tackle challenges faster, make smarter decisions, and rethink how work gets done. But not all AI works the same way and without a trusted model, things can go south very quickly.
Take Zillow’s $880 million misstep as a cautionary tale. On paper, Zillow’s AI-driven home-buying program seemed like a brilliant way to estimate home values. But behind the scenes? Bad data, blind spots, and lack of clarity turned this smart idea into a financial disaster.
So how do you build AI that delivers real, trustworthy insights? More importantly, which approach can help you keep your AI fair, ethical, and context-aware?
The promise of human-in-the-loop is paving the way toward responsible AI usage. In this article, we’ll examine how exactly this approach works—discussing core processes and exploring the many benefits.
Table of contents:
Human-in-the-loop (HITL) is a collaborative approach that integrates human judgment and expertise into AI development and decision-making processes. By actively participating in the training and operation of AI models, we can provide critical feedback, refine outputs, and correct biases. This continuous oversight improves model performance and helps align your AI with real-world complexities and ethical considerations.
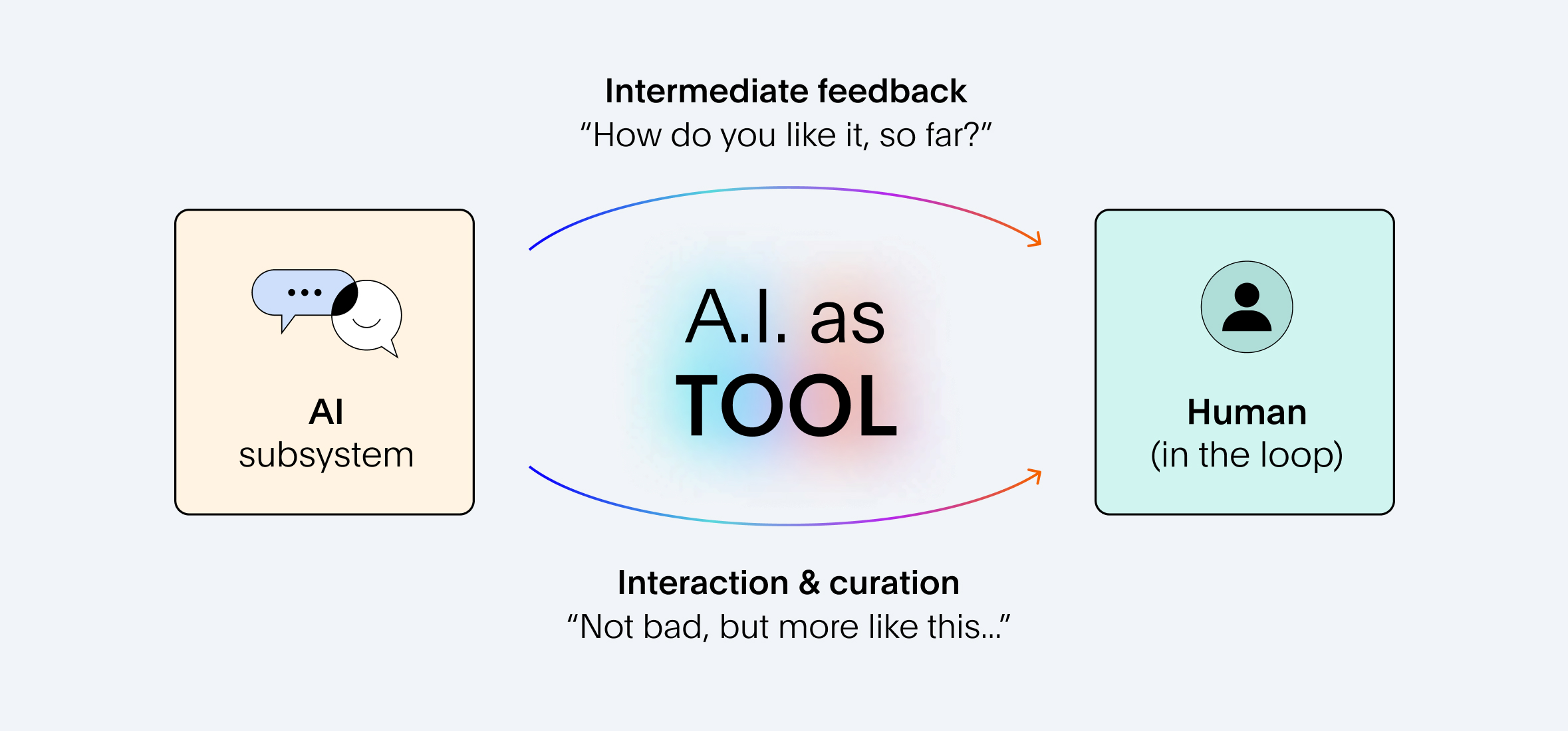
At this point, we all know AI—analyzing vast datasets, recognizing patterns, and even automating complex tasks. But here’s the catch: it struggles with nuance. Edge cases and real-world complexity can throw even the most powerful AI agent off track.
Here’s how integrating human oversight into AI processes can help you solve these challenges:
Human-guided data training: Instead of AI learning purely from raw, unlabeled data, you can step in to provide more structure and clarity. By labeling datasets, adding annotations, and categorizing text, you can reduce inconsistencies and offer the model the context it needs to deliver more meaningful outcomes.
Continuous feedback loops: You can provide feedback through two primary methods:
Active learning: AI models don’t just seek any input; they specifically ask for human guidance on uncertain or challenging data points to improve learning efficiency.
Reinforced learning: While this is a trial-and-error process, AI systems don’t just learn randomly—they follow a structured approach where they interact with an environment and optimize actions based on human-influenced reward signals.
That said, here are common ways you can create feedback loops:
Real-time error correction: By spotting and fixing errors in AI outputs immediately, you're allowing the model to learn from its missteps before it snowballs into bigger issues later. This reduces blind spots and helps the model become more accurate with each iteration.
Refining training data: Continuously feeding the model fresh and diverse data can help you fill contextual gaps that might’ve been overlooked in initial training. This process helps the model stay relevant and adaptable, allowing it to recognize new trends and enrich your data.
Bias detection: While powerful, AI isn't immune to hallucinations. Left unchecked, biases and anomalies can easily creep in, distorting decision-making. To keep your AI agents sharp and reliable, it's crucial to flag biases, detect anomalies, and adjust its parameters for optimal performance.
Setting ethical safeguards: Human feedback and collaboration can help leaders set ethical safeguards for responsible AI use. It allows you to define clear boundaries for acceptable pretraining data and prevent the inclusion of harmful or toxic content.
Intervention in critical scenarios: When AI encounters ambiguity, it doesn’t always know how to course-correct. That’s why human oversight becomes crucial. By scoring the model’s outputs, validating them, and fine-tuning LLM responses, you can guide AI algorithms through complex edge cases, helping them make more accurate and context-aware decisions.
With an active feedback loop, you’re not just improving outputs, you’re also cultivating a culture where people can trust and act on their data. Here’s why adopting this approach is critical for your business’ success:
Establishing transparency
For most business users, AI systems are ‘black boxes’ often shrouded in mystery. Sure, they may use the tools for everyday tasks, but the bigger question is: How can users rely on them for more complex, high-stakes decisions? Without transparency into how AI models process data and generate insights, it’s difficult to know if the results are built on solid data or if hidden biases and errors are creeping in.
With human oversight, you open this ‘black box’ and help stakeholders understand the logic and reasoning behind each output. This transparency helps users understand how these models work, and most importantly, why they behave the way they do.
Reducing instances of biases and hallucinations
Imagine this: You turn to LLMs to refine your audience targeting strategy. But when the results come in, you’re left scratching your head—your AI confidently reports that your target customers are concentrated in a region where your products aren’t even available.
This is a classic example of AI hallucinations. And it’s not just about inaccurate outputs—AI can also introduce privacy concerns, biases, and governance issues, making real-time decision-making risky.
The good news? With human-in-the-loop intervention, you can stop these errors before they spiral. By flagging anomalies, correcting inconsistencies, and filling in critical gaps—like regional availability, you can train your AI models to remain fair and ethical.
Improving adaptability to real-world scenarios
Without proper training and oversight, AI models may struggle to adapt to real-world complexities, leading to inaccurate or biased outputs. By training LLMs on diverse datasets and supplementing them with rare human knowledge, you improve their ability to generate relevant and context-aware content across different domains.
For example, suppose your company wants to forecast demand for a new, niche product with limited historical sales data. In this case, AI might struggle to make accurate predictions. However, by incorporating human expertise—like input from market analysts who understand the broader consumer trends—the model can be trained on more accurate insights.
2025 is set to be the year where businesses scale AI across every facet of their operations. However, to truly capitalize on these opportunities, your AI systems need more than just data—they need human judgment and expertise.
Here are a few examples where incorporating human-in-the-loop processes can make a game-changing difference:
Healthcare industry: AI systems are incredibly powerful tools for analyzing vast amounts of patient data and detecting early signs of common conditions like cancer and heart disease. However, when it comes to spotting rare diseases or complex cases, AI can’t connect the dots alone. That's where doctors and specialists come in. They can validate AI predictions, add crucial context, and refine the system based on their clinical expertise, guiding the agent toward a more effective treatment path.
Finance industry: Fraud detection is a key application of AI in finance, but some fraudulent activities are so sophisticated that algorithms might struggle to catch them. However, with proper feedback loops, this gap can be bridged. For instance, ThoughtSpot’s AI Agent, Spotter can instantly detect anomalies and send real-time alerts, empowering you to take swift action. This proactive approach ensures you can review and investigate suspicious transactions before they escalate into larger issues. You can also coach Spotter by providing feedback on flagged transactions.
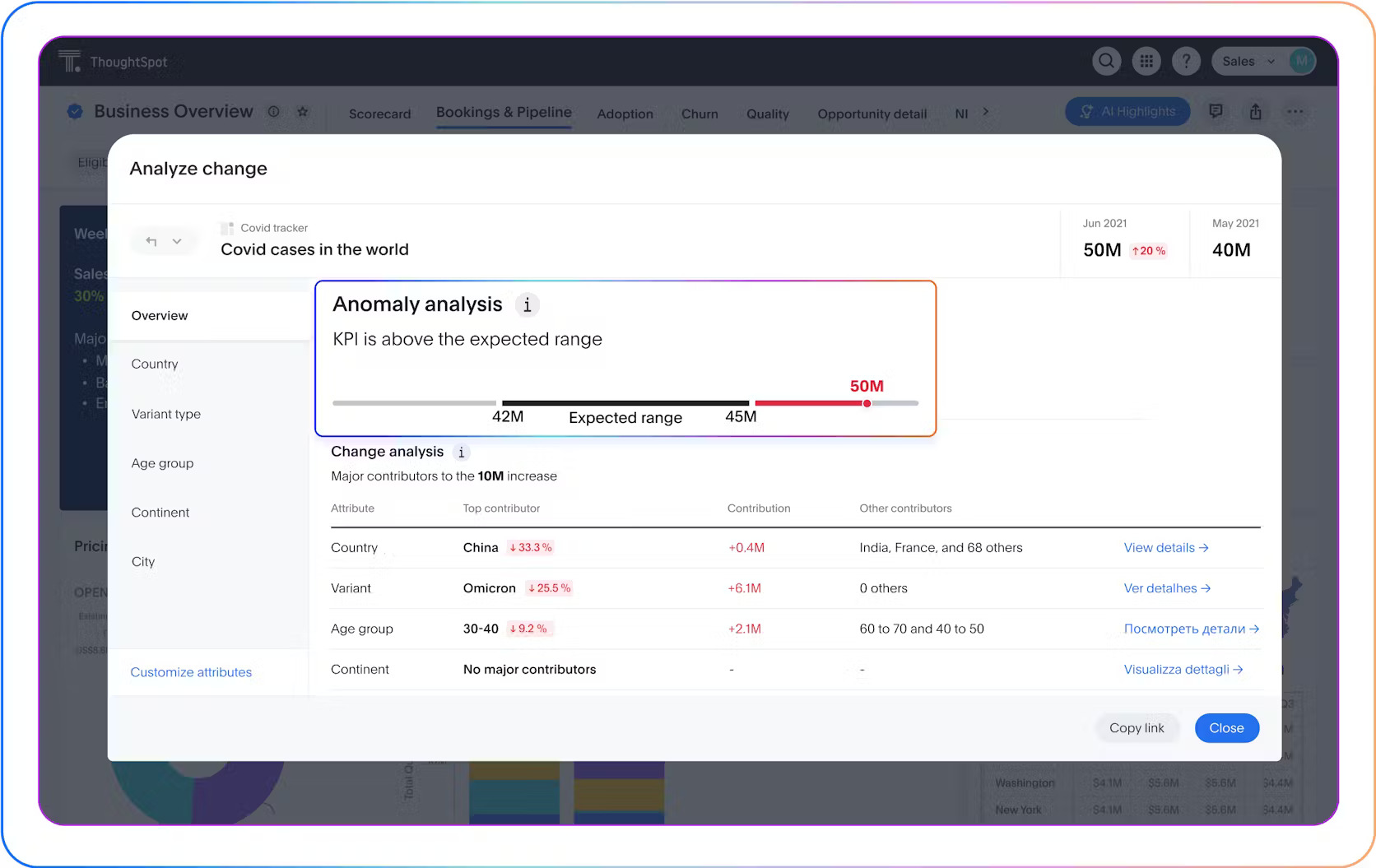
Manufacturing industry: By analyzing machinery data, AI can forecast when certain equipment is likely to fail, allowing companies to fix problems before they become costly. But what about unforeseeable events? This is where human expertise becomes invaluable. Skilled technicians can step in to assess the situation, validate AI predictions, and apply their experience to help the AI system deliver accurate and context-rich results.
The broader takeaway isn’t ‘How do we design more intelligent AI machines?’, but rather, ‘How do we leverage AI for better business outcomes?’. Because at the end of the day, AI is just a tool—the real power lies in how you apply it. To truly make an impact, you need an AI partner that:
Delivers trusted insights that are transparent, explainable, and understandable to any user, no matter their technical capabilities
Empowers data teams to refine AI’s understanding of key definitions and concepts
Gives administrators full control over who can train, oversee, and manage AI, with clear visibility into its performance
Meet Spotter—your trusted AI partner. With Spotter as your AI analyst, you’re gaining contextual insights that actually help you drive better decisions and outcomes.
Unlike other AI solutions, Spotter uses advanced semantic models and human-in-the-loop feedback to master your business language, delivering precise AI insights you can trust, no matter what industry you work in. Even better, Spotter’s underlying relational search engine ensures transparency by explaining answers in simple, accessible terms that anyone can understand—no complex SQL queries required.

Want to see how ThoughtSpot bridges the gap between people, data, and AI? Sign up for a ThoughtSpot demo today!