The world of Analytics is no different than any other technology-based solution domain in that the receptiveness of the audience to change is one of the most important factors impacting success. Tom Davenport’s book, Competing on Analytics, published in 2007, addressed this notion early on in the formalization of Analytics as a specific domain (even though most companies had been doing this kind of work for decades without calling it out specifically). Davenport published an analytics maturity model which addressed 5 key areas of measuring organizational capability in analytics, and thus potential for success: Data, Enterprise, Leadership, Targets, Analysts. While Data and Analysts are obvious topics of focus, Enterprise, Leadership, and Targets are often forgotten as an organization tries to embed analytics capabilities into their business processes. For the past decade, I have held roles focused on driving Analytics into the business, and have experienced first-hand the accuracy at which Davenport described the maturation process. Early in our Analytics journey at Caterpillar, we rapidly deployed predictive models at a few target areas of the business, only to be outright rejected. Working in a largely relationship based business, some very common excuses emerged as to why the outputs from predictive models were not accepted and insights not acted upon, including “the data is wrong” and “I know my customer/dealer/supplier.”
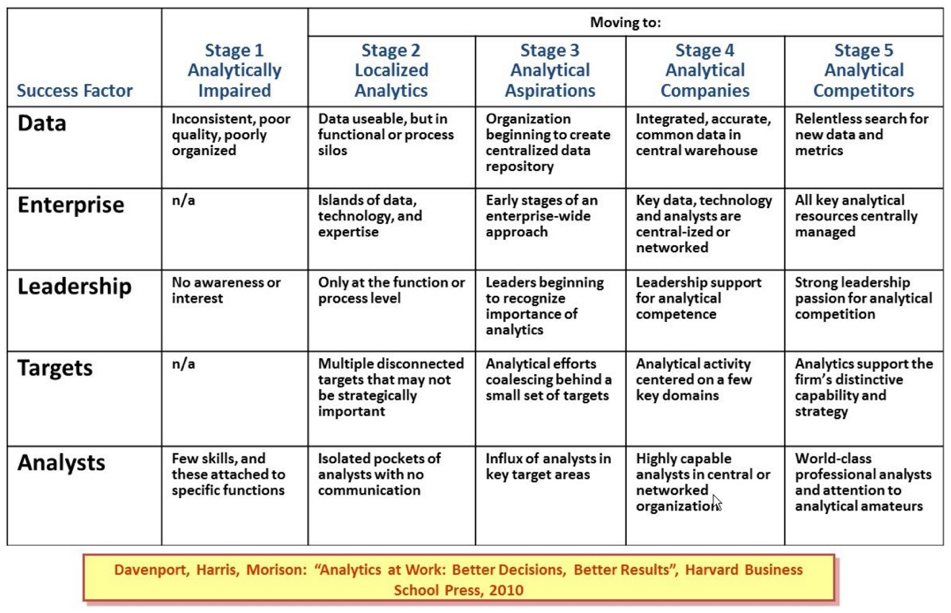
Just as Davenport’s model suggests, the transition from being data-centric, to KPI-centric, to adopting advanced analytics capabilities is in fact a step-by-step process. Skipping any step in that journey will likely lead to failure. In the instance of our failed predictive models from ten years ago, those very same models are now used every day, and generating significant value. The first hill to conquer in that journey was to gain accountability for the quality of the data within the business community. It is very easy for the business to point the finger at the Information Technology organization when dirty data is prevalent, but most of us understand that the root cause of dirty data is much more likely to be found in a lack of process discipline. Gaining accountability for the process discipline and thus the data that is output from that process is a leadership challenge … and a potentially daunting one at that. Establishing KPI’s for each line of business, and automated reporting of data against those KPI’s is one effective way to drive such accountability. Leaders need to be staunch in their position that the data isn’t wrong in this scenario. Over time, business users will become accustomed to “their data” and how it shows they are progressing towards “their goals,” and a data-driven culture can emerge. This is the progression through Davenport’s Stage 3 in Analytic Maturity.
As we look back at our own journey, we marvel at the amount of innovation and disruption that has occurred in the technologies that are available to facilitate this journey. From Data storage techniques that were analogous to a prison, to the emergence and acceptance of data marts, to the new concepts of data lakes. From developing reports in a very cumbersome and complex Business Intelligence environment in our early days, to the next generation of business intelligence capabilities that dramatically reduced the time and cost of reporting, to the Business User Self-Service model emerging now, it is sufficient to say that the investment in time, dollars, and resources to drive analytics into your business are far less today than ten years ago. Open source capabilities for both statistical modeling as well as visualization are not only common, but continuing to evolve. While available talent to do this kind of work is still below demand, the number of universities offering such coursework is exploding—though buyer beware. A common question from analytically immature organizations is now “how do I accelerate this journey,” and there are plenty of consultants and technology vendors waiting to answer that question for you. In the end, the real answer to that question lies in simply understanding how to walk your organization through a couple—seemingly simple—steps of change:
Data warehousing/standards to democratize your data to the technical community
Data Visualization capabilities to democratize your data to the business community and
KPI’s that measure the results of those business users (and those users accepting accountability for the quality of their data).
Then and only then will your organization be able to achieve the significant value potential a Data Scientist can bring through predictive models. Your investment decisions should be grounded in how they will help enable these steps, and knowing that without strong leadership support and key business targets for success, you will not likely get a positive return on that investment.
As you plan to drive Analytics into your business, take some time to understand the Davenport Analytics Maturity Model, and more importantly, those areas of Leadership, Enterprise, and Targets. Your likelihood for success will increase predictably!