Artificial Intelligence (AI) is the talk of the town, so it’s no surprise the newest wave of business intelligence (BI) solutions are harnessing this technology. In fact, our recent survey with MIT SMR Connections shows that 67% of leaders are using GenAI for data and analytics. Even better, they are seeing results: differentiation, revenue growth, and increased customer engagement just to name a few.
But what exactly can AI in BI can achieve and, more importantly, how can it drive outcomes for your business? Below, we’ve listed some of the top ways leaders are sparking their data renaissance with AI and how you can do the same for your business.
Table of contents:
We know BI is the use of technology to analyze and transform data into actionable insights. It usually includes leveraging BI tools to handle tasks like data collection, analysis, visualization, and interpretation. However, as the volume and complexity of data increases, businesses often struggle to uncover patterns, trends, or insights within their data. That’s where AI comes into play.
With AI-powered BI solutions, you take your analytical capabilities one step further. These solutions can sift through large datasets in real-time and identify patterns, correlations, and anomalies. Powerful capabilities like natural language processing (NLP), machine learning (ML), and deep learning can help you create powerful data models to predict outcomes or uncover hidden insights. Let’s look at some of the ways where AI-powered solutions are enabling end users to understand their business better and not just query their data:
1. Empowering self-service analytics
Earlier, non-technical users often faced lengthy delays in decision-making—sometimes waiting weeks or months—because they relied on reports from their data teams. When time is money, it’s beneficial to empower business users to quickly get the data insights they need so they can make timely, informed decisions. And this is why self-service analytics is crucial.
Here’s what David Stodder, former Senior Director of Research for BI at TDWI, says on the matter: “Self-service is a priority because it breaks [users] off from being completely dependent on IT and IT developers.”
He adds that data democratization entails adopting AI analytics tools that are more personalized to users’ needs in terms of search, analysis, and visualization.
Now with AI-Powered Analytics solutions like ThoughtSpot, business users can query data the same way they’d search for information on their favorite search engine. Here’s one real-life example of self-service analytics in action: Using ThoughtSpot’s natural language search, Wellthy’s care team could visualize real-time patient data, filter and drill into specific searches, and segment members based on their specific needs—without relying on their data teams. Thanks to ThoughtSpot, even Wellthy’s data teams felt liberated to work on high-value initiatives, saving the company over $200k by increasing analyst efficiency.
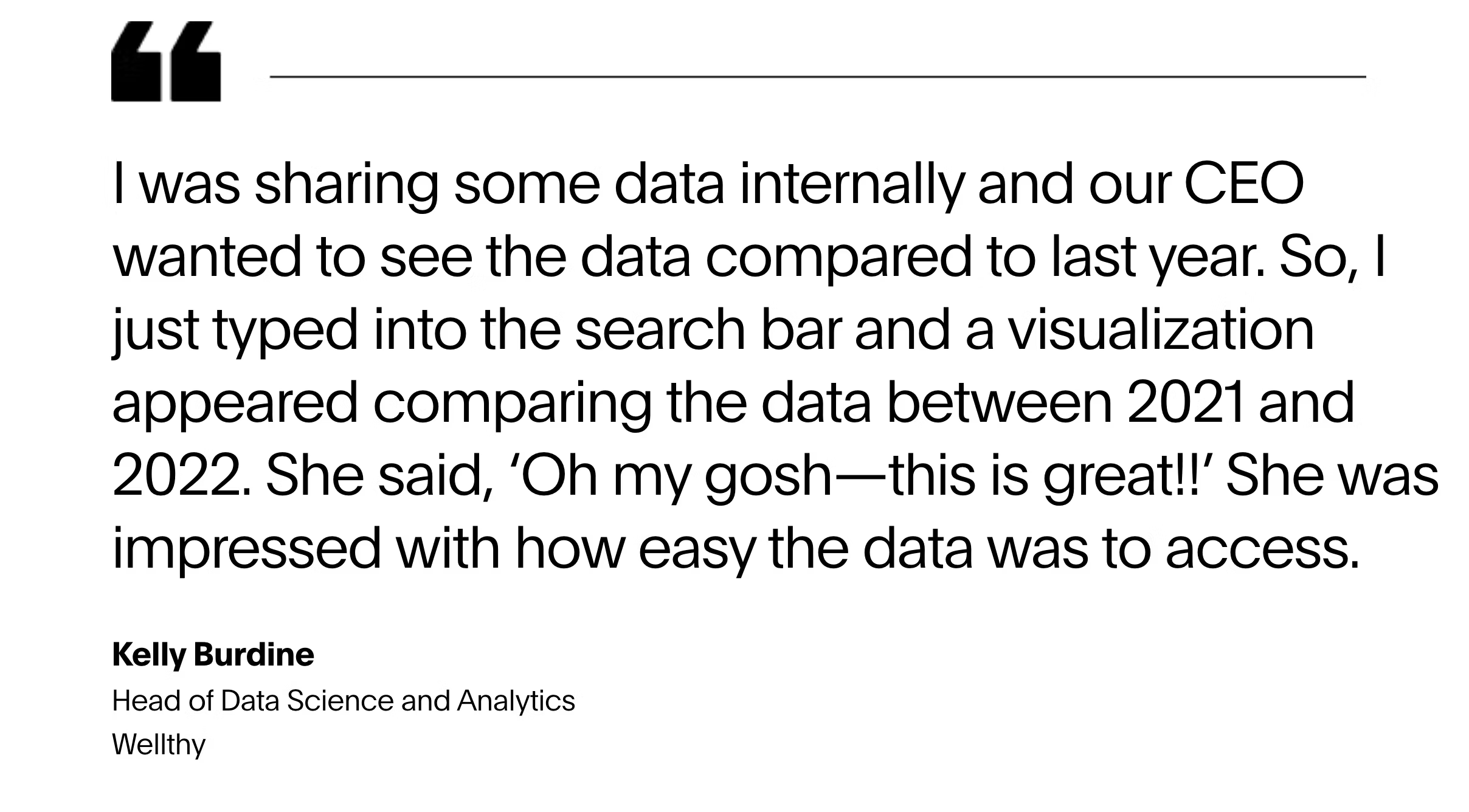
💡Pro tip: If you haven’t already launched a data fluency and AI training program, do so now. Create customized learning programs that help users understand how to work with their new BI tools and drive their own strategic decisions.
2. Crafting better data narratives
When you’re making strategic business decisions, you’ll need to be able to zoom in and out of your data to get a complete picture. With modern AI-powered dashboard solutions like ThoughtSpot, organizations are no longer stuck analyzing past data. They can monitor KPI changes in real-time, predict future trends, and uncover unusual patterns—all with just a few clicks. Armed with this information, you can craft compelling data stories, ensuring you can communicate important insights to all stakeholders.
For instance, instead of presenting just a static chart showcasing your sales performance, you can create interactive dashboards that allow you to drill down into specific data points, compare past and present performance, and highlight important trends. Such narratives can significantly enhance decision-making, offering stakeholders a clearer view of potential improvements and challenges.
💡Pro tip: Ask yourself, does your current BI tool allow you to tailor your visualizations? Does it support drill-anywhere visualizations that help you explore specific data points, categories, and time periods? If the answer is no, it’s time to invest in other tools.
3. Augmented intelligence: Less plumbing, faster insights
Finding the "aha!" moments in your data requires a lot of manual plumbing and processing. It is vital to consistently ensure the data you feed into your analytics systems is readily accessible, clean, and reliable. However, such activities can take up half the time of your data team, shifting their focus away from value-added activities.
Luckily, with the emergence of cloud computing and GenAI, analysts can create a single source of truth and democratize analytics for all. The entire process of data transformation and even data modeling can be made easier and faster with AI. However, human oversight remains crucial.
That’s why adopting the right augmented intelligence solution matters. For instance, ThoughtSpot was the first to ride the AI wave back in the year 2023 and is now recognized as a leader in the 2024 Gartner MQ for Analytics and BI. What sets ThoughtSpot apart is its promise to offer enterprise-grade accuracy and governance. As a matter of fact, customers report up to 95% accuracy when using ThoughtSpot Spotter compared to GPT alone. This level of accuracy and precision is possible because of advanced human-in-the-loop feedback controls that allow you to improve LLM outputs.
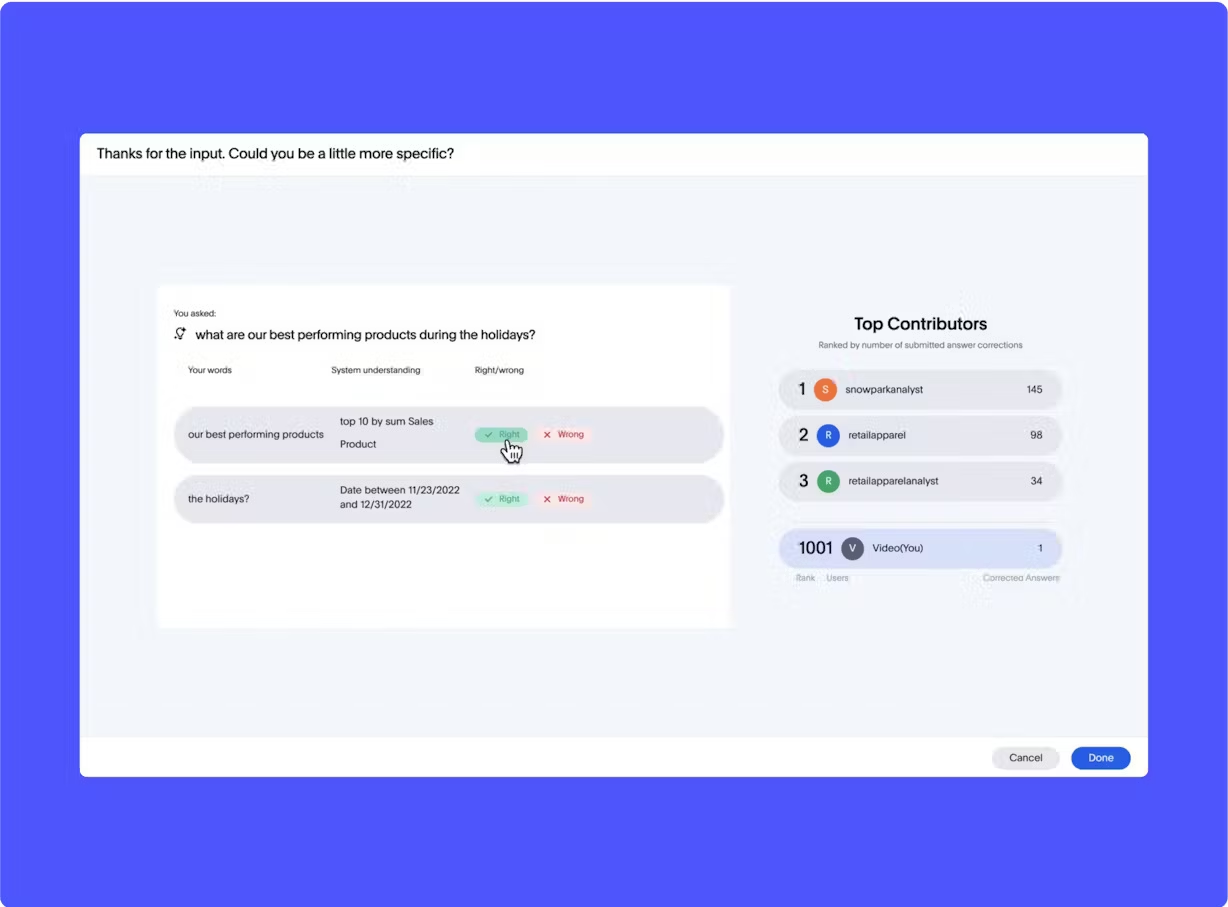
💡Pro tip: Prioritize BI solutions that allow for a human-in-the-loop feedback feature so your AI model can provide accurate, business-specific answers.
4. Paving the way for advanced analytics
Of all the emergent benefits of AI in BI, predicting outcomes is perhaps the most intriguing. By leveraging ML algorithms and advanced data modeling techniques, AI can help you build complex predictive models that create forecasts or provide recommendations based on historical data.
For instance, AI in business analytics can help retailors predict inventory needs based on past purchasing behaviors and seasonal trends. Similarly, healthcare providers can forecast patient admission rates by analyzing historical patient data. This ability allows organizations to meet future demands and innovate new offerings, giving them a competitive edge.
💡Pro tip: While predictive analytics is certainly exciting, implement a risk mitigation plan to reduce the chances of data hallucinations and biases.
Without a robust, repeatable, and scalable data value chain, scaling AI-powered analytics becomes nearly impossible. To help you avoid common pitfalls, here are the key challenges every organization must address when applying AI in BI:
1. Ensuring data quality
Research by Gartner shows only 4% of IT leaders say their data is AI-ready. Issues such as inconsistent data entry, missing or incorrect values, and irrelevant information can significantly undermine the accuracy of your AI models. With bad data, even organizations with mature business intelligence initiatives can experience instances of hallucinations and biases, resulting in confusion and misinformed decision-making. Also, when your users do not trust the data, it doesn't matter if you have empowered them to analyze the data themselves with self-service analytics tools. They simply won’t adopt them.
That’s why data cleaning and transformation are critical in getting the most value possible from your AI-powered data stack. Organizations must have a holistic strategy focused on data integrity and compliance. Key principles of this strategy should include rigorous data standardization, seamless connectivity with diverse data sources, and continuous monitoring of AI systems. These efforts ensure AI systems are not only industry-compliant but also capable of making decisions that are accurate and ethically sound.
2. The black box problem
AI-fueled insights can help business users take action to the point of impact. However, in many cases, there is a lack of transparency and explainability in how AI models work and arrive at a decision. Without visibility into how decisions are made, it becomes harder to detect biases in the model.
This is why, it is more important than ever to seek AI-powered BI solutions that have a built-in mechanism for users that helps them continuously monitor the model’s performance. It should also allow you to run appropriate tests to reduce or eliminate biases in their models.
For instance, ThoughtSpot has the BI industry’s most advanced human-in-the-loop feedback (HITL) capabilities that center human knowledge, business context, and security to build trust and accuracy across the organization. Even, data admins can further curate this feedback into a business-specific glossary, evolving into stewards of organizational intelligence. This collaborative approach improves model accuracy and builds a foundation of trust and reliability.

3. Skills gaps
The successful implementation of AI in BI requires a workforce equipped with the right skills to manage and leverage this technology. However, research by Forbes shows that while 75% of companies are adopting AI, only 35% of employees have received AI training in the past year. This highlights a significant skills gap, with many users lacking expertise in critical areas such as machine learning, data engineering, and AI ethics.
To bridge this gap, organizations must adopt faster, more inclusive skilling approaches to ensure users with varying levels of expertise can keep up with rapidly evolving AI technologies. Additionally, organizations must address users' concerns and ensure their talent is well-prepared and confident in using AI-powered tools.
4. Ethical concerns
AI-driven attacks and misinformation can have far-reaching impacts, damaging an organization's reputation, hindering productivity, and undermining its ability to respond effectively. Gartner research shows that 50% of consumers say they do not trust that humans will use AI responsibly, highlighting the growing concern over the ethical use of AI.
For organizations, it’s essential that customers feel confident in the use of AI systems, especially since regulators impose stricter compliance rules around AI ethics and data usage. While these challenges also exist with traditional BI systems, the increased autonomy of AI tools makes the need for implementing robust security controls even more urgent.
AI in BI is being applied across a wide range of industries and business functions. Here are a few examples of how organizations are using AI to drive significant outcomes for their businesses:
1. Customer-focused
AI algorithms can help your business analyze historical customer data, sales trends, and other relevant factors for segmentation, churn prediction, and personalization.
Picture this: As an e-commerce retailer, you notice a significant drop in sales performance in a specific region. Concerned about customer satisfaction, you look at the data to get to the root of this problem. Traditional BI may offer static snapshots, such as sales trends or regional performance comparisons. While these insights are helpful, they lack the depth to truly explain why the problem exists.
By adopting an AI-powered solution likeThoughtSpot, you can go beyond surface-level data. With Spotter, as your AI Analyst, you can immediately gain AI insights and real-time visualizations to identify the places where you can make the most impact.
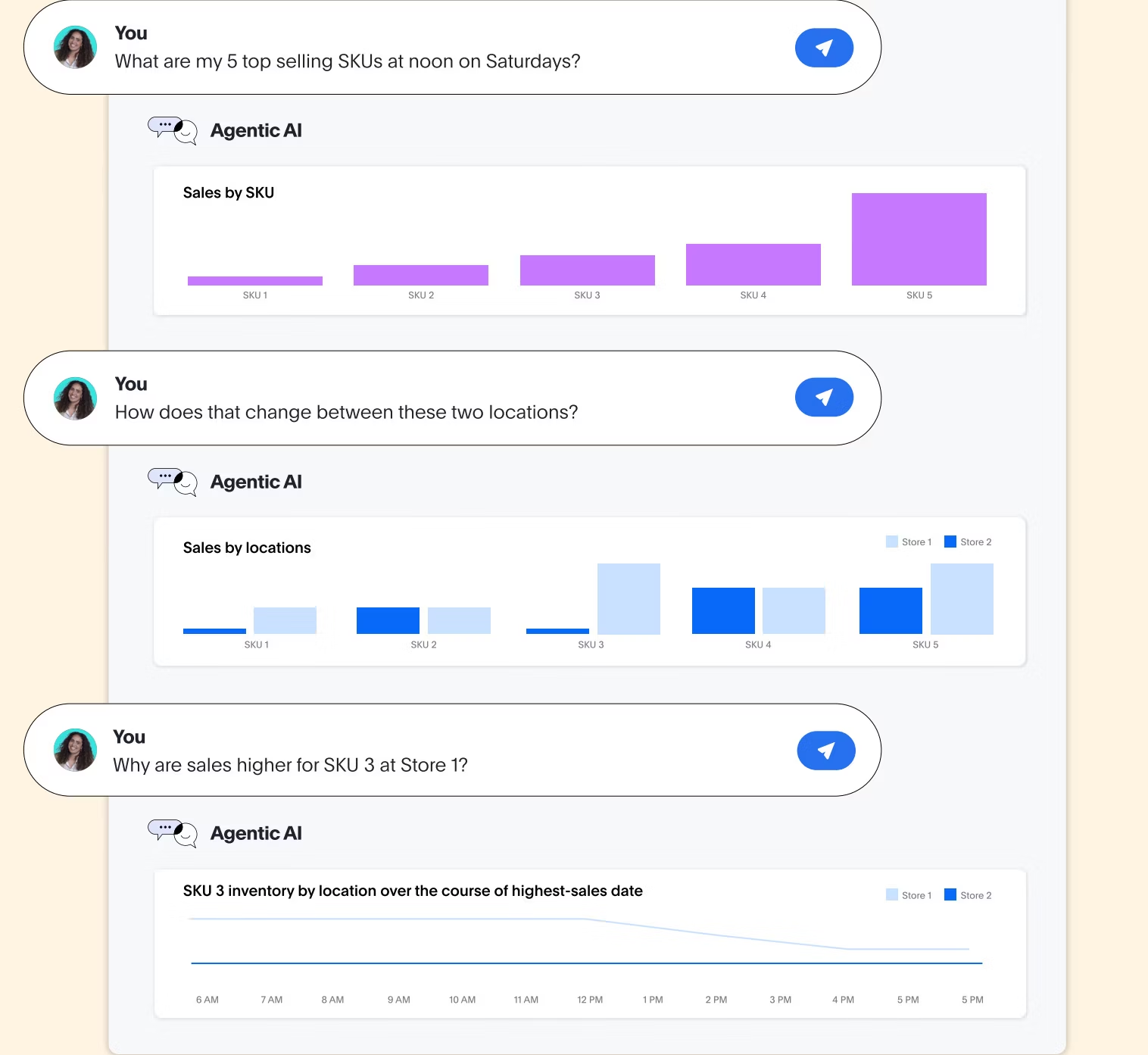
With these insights, you can pinpoint the exact issues behind your sales performance—whether it’s delayed shipments, product stockouts, or shifting customer preferences. This clarity enables you to take targeted actions like offering tailored promotions to specific customer segments, adjusting inventory levels for high-demand products, or optimizing your shipping processes to reduce delays.
2. Person-focused
AI-powered analytics can help your business teams manage various operational challenges such as predicting support needs, managing risks, and enhancing customer retention strategies.
Take this example: Let’s take the healthcare industry for instance. By tracking the readmission rate of patients and deploying AI algorithms, healthcare professionals can identify the root cause behind recent readmissions and take corrective actions. This in-depth analysis will enable organizations to assess the efficiency of their care programs, leading to a reduction in unnecessary healthcare costs and better healthcare outcomes for consumers.
3. Risk and fraud focused
Financial data fuels growth and success for any business. But it also exposes businesses to risks such as fraud, errors, and compliance issues. To mitigate these risks, finance teams can use AI-powered analytics to analyze transactions, identify suspicious patterns, and flag potentially fraudulent activities in real-time.
Picture this: Imagine you're a financial analyst tasked with overseeing corporate spending across different regions. Reviewing expense reports manually can be tedious, time-consuming, and prone to human error, making it easy for irregularities to slip through cracks.
With an AI-powered analytics solution like ThoughtSpot, you can leverage SpotIQ to perform ‘Anamoly analysis’. This powerful tool automatically scans your data, identifying outliers and unusual patterns that may indicate issues, such as unexpected spikes in spending or discrepancies in vendor transactions. Doing so, allows you to address these issues before they escalate, saving time and reducing risk.
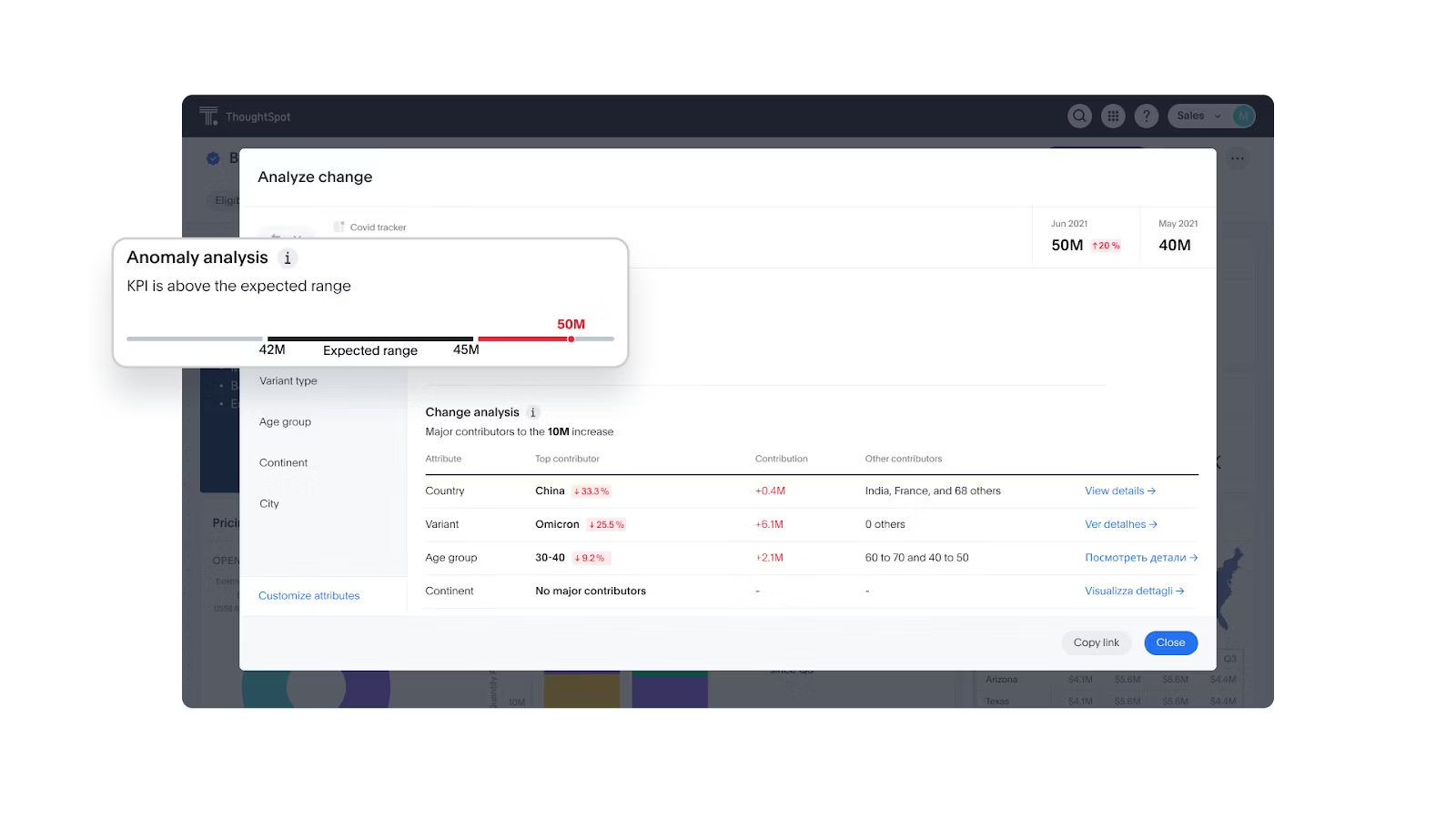
4. Supply chain focused
Managing global supply chains can be complex for businesses, especially when raw material prices and availability are constantly fluctuating. With AI-powered analytics, you can decrease unnecessary procurement costs by understanding fluctuations in your budget variance and spending patterns. This detailed analysis will help you monitor how closely the actual procurement spending aligns with the budgeted amounts and indicates areas where your procurement activities are over budget.
Here’s how ThoughtSpot SpotIQ auto-analyzes your spending and detects real-time anomalies in data, reducing operational overheads and boosting efficiency:
While the race to the “AI gold rush” rages on, it is important not to get distracted by flashy BI solutions. Researching and gaining hands-on experience with different GenAI products is crucial to understanding trust, accuracy, and cost trade-offs.
Even though ThoughtSpot stands out as a true leader among legacy BI solutions, we still encourage you to take an interactive product tour. This firsthand experience will demonstrate how ThoughtSpot can turn data into insights and drive meaningful outcomes across your business.